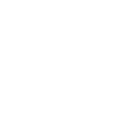
使用Player FM应用程序离线!
Decompiling Dreams: A New Approach to ARC? - Alessandro Palmarini
Manage episode 445907383 series 2803422
Alessandro Palmarini is a post-baccalaureate researcher at the Santa Fe Institute working under the supervision of Melanie Mitchell. He completed his undergraduate degree in Artificial Intelligence and Computer Science at the University of Edinburgh. Palmarini's current research focuses on developing AI systems that can efficiently acquire new skills from limited data, inspired by François Chollet's work on measuring intelligence. His work builds upon the DreamCoder program synthesis system, introducing a novel approach called "dream decompiling" to improve library learning in inductive program synthesis. Palmarini is particularly interested in addressing the Abstraction and Reasoning Corpus (ARC) challenge, aiming to create AI systems that can perform abstract reasoning tasks more efficiently than current approaches. His research explores the balance between computational efficiency and data efficiency in AI learning processes.
DO YOU WANT WORK ON ARC with the MindsAI team (current ARC winners)? MLST is sponsored by Tufa Labs: Focus: ARC, LLMs, test-time-compute, active inference, system2 reasoning, and more. Future plans: Expanding to complex environments like Warcraft 2 and Starcraft 2. Interested? Apply for an ML research position: benjamin@tufa.ai
TOC:
1. Intelligence Measurement in AI Systems
[00:00:00] 1.1 Defining Intelligence in AI Systems
[00:02:00] 1.2 Research at Santa Fe Institute
[00:04:35] 1.3 Impact of Gaming on AI Development
[00:05:10] 1.4 Comparing AI and Human Learning Efficiency
2. Efficient Skill Acquisition in AI
[00:06:40] 2.1 Intelligence as Skill Acquisition Efficiency
[00:08:25] 2.2 Limitations of Current AI Systems in Generalization
[00:09:45] 2.3 Human vs. AI Cognitive Processes
[00:10:40] 2.4 Measuring AI Intelligence: Chollet's ARC Challenge
3. Program Synthesis and ARC Challenge
[00:12:55] 3.1 Philosophical Foundations of Program Synthesis
[00:17:14] 3.2 Introduction to Program Induction and ARC Tasks
[00:18:49] 3.3 DreamCoder: Principles and Techniques
[00:27:55] 3.4 Trade-offs in Program Synthesis Search Strategies
[00:31:52] 3.5 Neural Networks and Bayesian Program Learning
4. Advanced Program Synthesis Techniques
[00:32:30] 4.1 DreamCoder and Dream Decompiling Approach
[00:39:00] 4.2 Beta Distribution and Caching in Program Synthesis
[00:45:10] 4.3 Performance and Limitations of Dream Decompiling
[00:47:45] 4.4 Alessandro's Approach to ARC Challenge
[00:51:12] 4.5 Conclusion and Future Discussions
Refs:
Full reflist on YT VD, Show Notes and MP3 metadata
217集单集
Manage episode 445907383 series 2803422
Alessandro Palmarini is a post-baccalaureate researcher at the Santa Fe Institute working under the supervision of Melanie Mitchell. He completed his undergraduate degree in Artificial Intelligence and Computer Science at the University of Edinburgh. Palmarini's current research focuses on developing AI systems that can efficiently acquire new skills from limited data, inspired by François Chollet's work on measuring intelligence. His work builds upon the DreamCoder program synthesis system, introducing a novel approach called "dream decompiling" to improve library learning in inductive program synthesis. Palmarini is particularly interested in addressing the Abstraction and Reasoning Corpus (ARC) challenge, aiming to create AI systems that can perform abstract reasoning tasks more efficiently than current approaches. His research explores the balance between computational efficiency and data efficiency in AI learning processes.
DO YOU WANT WORK ON ARC with the MindsAI team (current ARC winners)? MLST is sponsored by Tufa Labs: Focus: ARC, LLMs, test-time-compute, active inference, system2 reasoning, and more. Future plans: Expanding to complex environments like Warcraft 2 and Starcraft 2. Interested? Apply for an ML research position: benjamin@tufa.ai
TOC:
1. Intelligence Measurement in AI Systems
[00:00:00] 1.1 Defining Intelligence in AI Systems
[00:02:00] 1.2 Research at Santa Fe Institute
[00:04:35] 1.3 Impact of Gaming on AI Development
[00:05:10] 1.4 Comparing AI and Human Learning Efficiency
2. Efficient Skill Acquisition in AI
[00:06:40] 2.1 Intelligence as Skill Acquisition Efficiency
[00:08:25] 2.2 Limitations of Current AI Systems in Generalization
[00:09:45] 2.3 Human vs. AI Cognitive Processes
[00:10:40] 2.4 Measuring AI Intelligence: Chollet's ARC Challenge
3. Program Synthesis and ARC Challenge
[00:12:55] 3.1 Philosophical Foundations of Program Synthesis
[00:17:14] 3.2 Introduction to Program Induction and ARC Tasks
[00:18:49] 3.3 DreamCoder: Principles and Techniques
[00:27:55] 3.4 Trade-offs in Program Synthesis Search Strategies
[00:31:52] 3.5 Neural Networks and Bayesian Program Learning
4. Advanced Program Synthesis Techniques
[00:32:30] 4.1 DreamCoder and Dream Decompiling Approach
[00:39:00] 4.2 Beta Distribution and Caching in Program Synthesis
[00:45:10] 4.3 Performance and Limitations of Dream Decompiling
[00:47:45] 4.4 Alessandro's Approach to ARC Challenge
[00:51:12] 4.5 Conclusion and Future Discussions
Refs:
Full reflist on YT VD, Show Notes and MP3 metadata
217集单集
所有剧集
×欢迎使用Player FM
Player FM正在网上搜索高质量的播客,以便您现在享受。它是最好的播客应用程序,适用于安卓、iPhone和网络。注册以跨设备同步订阅。