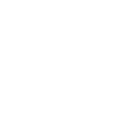
使用Player FM应用程序离线!
Want to Understand Neural Networks? Think Elastic Origami! - Prof. Randall Balestriero
Manage episode 465607840 series 2803422
Professor Randall Balestriero joins us to discuss neural network geometry, spline theory, and emerging phenomena in deep learning, based on research presented at ICML. Topics include the delayed emergence of adversarial robustness in neural networks ("grokking"), geometric interpretations of neural networks via spline theory, and challenges in reconstruction learning. We also cover geometric analysis of Large Language Models (LLMs) for toxicity detection and the relationship between intrinsic dimensionality and model control in RLHF.
SPONSOR MESSAGES:
***
CentML offers competitive pricing for GenAI model deployment, with flexible options to suit a wide range of models, from small to large-scale deployments.
https://centml.ai/pricing/
Tufa AI Labs is a brand new research lab in Zurich started by Benjamin Crouzier focussed on o-series style reasoning and AGI. Are you interested in working on reasoning, or getting involved in their events?
Goto https://tufalabs.ai/
***
Randall Balestriero
https://x.com/randall_balestr
https://randallbalestriero.github.io/
Show notes and transcript: https://www.dropbox.com/scl/fi/3lufge4upq5gy0ug75j4a/RANDALLSHOW.pdf?rlkey=nbemgpa0jhawt1e86rx7372e4&dl=0
TOC:
- Introduction
- 00:00:00: Introduction
- Neural Network Geometry and Spline Theory
- 00:01:41: Neural Network Geometry and Spline Theory
- 00:07:41: Deep Networks Always Grok
- 00:11:39: Grokking and Adversarial Robustness
- 00:16:09: Double Descent and Catastrophic Forgetting
- Reconstruction Learning
- 00:18:49: Reconstruction Learning
- 00:24:15: Frequency Bias in Neural Networks
- Geometric Analysis of Neural Networks
- 00:29:02: Geometric Analysis of Neural Networks
- 00:34:41: Adversarial Examples and Region Concentration
- LLM Safety and Geometric Analysis
- 00:40:05: LLM Safety and Geometric Analysis
- 00:46:11: Toxicity Detection in LLMs
- 00:52:24: Intrinsic Dimensionality and Model Control
- 00:58:07: RLHF and High-Dimensional Spaces
- Conclusion
- 01:02:13: Neural Tangent Kernel
- 01:08:07: Conclusion
REFS:
[00:01:35] Humayun – Deep network geometry & input space partitioning
https://arxiv.org/html/2408.04809v1
[00:03:55] Balestriero & Paris – Linking deep networks to adaptive spline operators
https://proceedings.mlr.press/v80/balestriero18b/balestriero18b.pdf
[00:13:55] Song et al. – Gradient-based white-box adversarial attacks
https://arxiv.org/abs/2012.14965
[00:16:05] Humayun, Balestriero & Baraniuk – Grokking phenomenon & emergent robustness
https://arxiv.org/abs/2402.15555
[00:18:25] Humayun – Training dynamics & double descent via linear region evolution
https://arxiv.org/abs/2310.12977
[00:20:15] Balestriero – Power diagram partitions in DNN decision boundaries
https://arxiv.org/abs/1905.08443
[00:23:00] Frankle & Carbin – Lottery Ticket Hypothesis for network pruning
https://arxiv.org/abs/1803.03635
[00:24:00] Belkin et al. – Double descent phenomenon in modern ML
https://arxiv.org/abs/1812.11118
[00:25:55] Balestriero et al. – Batch normalization’s regularization effects
https://arxiv.org/pdf/2209.14778
[00:29:35] EU – EU AI Act 2024 with compute restrictions
https://www.lw.com/admin/upload/SiteAttachments/EU-AI-Act-Navigating-a-Brave-New-World.pdf
[00:39:30] Humayun, Balestriero & Baraniuk – SplineCam: Visualizing deep network geometry
https://openaccess.thecvf.com/content/CVPR2023/papers/Humayun_SplineCam_Exact_Visualization_and_Characterization_of_Deep_Network_Geometry_and_CVPR_2023_paper.pdf
[00:40:40] Carlini – Trade-offs between adversarial robustness and accuracy
https://arxiv.org/pdf/2407.20099
[00:44:55] Balestriero & LeCun – Limitations of reconstruction-based learning methods
https://openreview.net/forum?id=ez7w0Ss4g9
(truncated, see shownotes PDF)
216集单集
Manage episode 465607840 series 2803422
Professor Randall Balestriero joins us to discuss neural network geometry, spline theory, and emerging phenomena in deep learning, based on research presented at ICML. Topics include the delayed emergence of adversarial robustness in neural networks ("grokking"), geometric interpretations of neural networks via spline theory, and challenges in reconstruction learning. We also cover geometric analysis of Large Language Models (LLMs) for toxicity detection and the relationship between intrinsic dimensionality and model control in RLHF.
SPONSOR MESSAGES:
***
CentML offers competitive pricing for GenAI model deployment, with flexible options to suit a wide range of models, from small to large-scale deployments.
https://centml.ai/pricing/
Tufa AI Labs is a brand new research lab in Zurich started by Benjamin Crouzier focussed on o-series style reasoning and AGI. Are you interested in working on reasoning, or getting involved in their events?
Goto https://tufalabs.ai/
***
Randall Balestriero
https://x.com/randall_balestr
https://randallbalestriero.github.io/
Show notes and transcript: https://www.dropbox.com/scl/fi/3lufge4upq5gy0ug75j4a/RANDALLSHOW.pdf?rlkey=nbemgpa0jhawt1e86rx7372e4&dl=0
TOC:
- Introduction
- 00:00:00: Introduction
- Neural Network Geometry and Spline Theory
- 00:01:41: Neural Network Geometry and Spline Theory
- 00:07:41: Deep Networks Always Grok
- 00:11:39: Grokking and Adversarial Robustness
- 00:16:09: Double Descent and Catastrophic Forgetting
- Reconstruction Learning
- 00:18:49: Reconstruction Learning
- 00:24:15: Frequency Bias in Neural Networks
- Geometric Analysis of Neural Networks
- 00:29:02: Geometric Analysis of Neural Networks
- 00:34:41: Adversarial Examples and Region Concentration
- LLM Safety and Geometric Analysis
- 00:40:05: LLM Safety and Geometric Analysis
- 00:46:11: Toxicity Detection in LLMs
- 00:52:24: Intrinsic Dimensionality and Model Control
- 00:58:07: RLHF and High-Dimensional Spaces
- Conclusion
- 01:02:13: Neural Tangent Kernel
- 01:08:07: Conclusion
REFS:
[00:01:35] Humayun – Deep network geometry & input space partitioning
https://arxiv.org/html/2408.04809v1
[00:03:55] Balestriero & Paris – Linking deep networks to adaptive spline operators
https://proceedings.mlr.press/v80/balestriero18b/balestriero18b.pdf
[00:13:55] Song et al. – Gradient-based white-box adversarial attacks
https://arxiv.org/abs/2012.14965
[00:16:05] Humayun, Balestriero & Baraniuk – Grokking phenomenon & emergent robustness
https://arxiv.org/abs/2402.15555
[00:18:25] Humayun – Training dynamics & double descent via linear region evolution
https://arxiv.org/abs/2310.12977
[00:20:15] Balestriero – Power diagram partitions in DNN decision boundaries
https://arxiv.org/abs/1905.08443
[00:23:00] Frankle & Carbin – Lottery Ticket Hypothesis for network pruning
https://arxiv.org/abs/1803.03635
[00:24:00] Belkin et al. – Double descent phenomenon in modern ML
https://arxiv.org/abs/1812.11118
[00:25:55] Balestriero et al. – Batch normalization’s regularization effects
https://arxiv.org/pdf/2209.14778
[00:29:35] EU – EU AI Act 2024 with compute restrictions
https://www.lw.com/admin/upload/SiteAttachments/EU-AI-Act-Navigating-a-Brave-New-World.pdf
[00:39:30] Humayun, Balestriero & Baraniuk – SplineCam: Visualizing deep network geometry
https://openaccess.thecvf.com/content/CVPR2023/papers/Humayun_SplineCam_Exact_Visualization_and_Characterization_of_Deep_Network_Geometry_and_CVPR_2023_paper.pdf
[00:40:40] Carlini – Trade-offs between adversarial robustness and accuracy
https://arxiv.org/pdf/2407.20099
[00:44:55] Balestriero & LeCun – Limitations of reconstruction-based learning methods
https://openreview.net/forum?id=ez7w0Ss4g9
(truncated, see shownotes PDF)
216集单集
所有剧集
×欢迎使用Player FM
Player FM正在网上搜索高质量的播客,以便您现在享受。它是最好的播客应用程序,适用于安卓、iPhone和网络。注册以跨设备同步订阅。