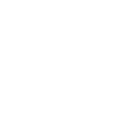
使用Player FM应用程序离线!
What’s the Magic Word? A Control Theory of LLM Prompting.
Manage episode 422128945 series 2803422
These two scientists have mapped out the insides or “reachable space” of a language model using control theory, what they discovered was extremely surprising.
Please support us on Patreon to get access to the private Discord server, bi-weekly calls, early access and ad-free listening.
https://patreon.com/mlst
YT version: https://youtu.be/Bpgloy1dDn0
Aman Bhargava from Caltech and Cameron Witkowski from the University of Toronto to discuss their groundbreaking paper, “What’s the Magic Word? A Control Theory of LLM Prompting.” (the main theorem on self-attention controllability was developed in collaboration with Dr. Shi-Zhuo Looi from Caltech).
They frame LLM systems as discrete stochastic dynamical systems. This means they look at LLMs in a structured way, similar to how we analyze control systems in engineering. They explore the “reachable set” of outputs for an LLM. Essentially, this is the range of possible outputs the model can generate from a given starting point when influenced by different prompts. The research highlights that prompt engineering, or optimizing the input tokens, can significantly influence LLM outputs. They show that even short prompts can drastically alter the likelihood of specific outputs. Aman and Cameron’s work might be a boon for understanding and improving LLMs. They suggest that a deeper exploration of control theory concepts could lead to more reliable and capable language models.
We dropped an additional, more technical video on the research on our Twitter account here: https://x.com/MLStreetTalk/status/1795093759471890606
Additional 20 minutes of unreleased footage on our Patreon here: https://www.patreon.com/posts/whats-magic-word-104922629
What's the Magic Word? A Control Theory of LLM Prompting (Aman Bhargava, Cameron Witkowski, Manav Shah, Matt Thomson)
https://arxiv.org/abs/2310.04444
LLM Control Theory Seminar (April 2024)
https://www.youtube.com/watch?v=9QtS9sVBFM0
Society for the pursuit of AGI (Cameron founded it)
https://agisociety.mydurable.com/
Roger Federer demo
http://conway.languagegame.io/inference
Neural Cellular Automata, Active Inference, and the Mystery of Biological Computation (Aman)
https://aman-bhargava.com/ai/neuro/neuromorphic/2024/03/25/nca-do-active-inference.html
Aman and Cameron also want to thank Dr. Shi-Zhuo Looi and Prof. Matt Thomson from from Caltech for help and advice on their research. (https://thomsonlab.caltech.edu/ and https://pma.caltech.edu/people/looi-shi-zhuo)
https://x.com/ABhargava2000
https://x.com/witkowski_cam
216集单集
Manage episode 422128945 series 2803422
These two scientists have mapped out the insides or “reachable space” of a language model using control theory, what they discovered was extremely surprising.
Please support us on Patreon to get access to the private Discord server, bi-weekly calls, early access and ad-free listening.
https://patreon.com/mlst
YT version: https://youtu.be/Bpgloy1dDn0
Aman Bhargava from Caltech and Cameron Witkowski from the University of Toronto to discuss their groundbreaking paper, “What’s the Magic Word? A Control Theory of LLM Prompting.” (the main theorem on self-attention controllability was developed in collaboration with Dr. Shi-Zhuo Looi from Caltech).
They frame LLM systems as discrete stochastic dynamical systems. This means they look at LLMs in a structured way, similar to how we analyze control systems in engineering. They explore the “reachable set” of outputs for an LLM. Essentially, this is the range of possible outputs the model can generate from a given starting point when influenced by different prompts. The research highlights that prompt engineering, or optimizing the input tokens, can significantly influence LLM outputs. They show that even short prompts can drastically alter the likelihood of specific outputs. Aman and Cameron’s work might be a boon for understanding and improving LLMs. They suggest that a deeper exploration of control theory concepts could lead to more reliable and capable language models.
We dropped an additional, more technical video on the research on our Twitter account here: https://x.com/MLStreetTalk/status/1795093759471890606
Additional 20 minutes of unreleased footage on our Patreon here: https://www.patreon.com/posts/whats-magic-word-104922629
What's the Magic Word? A Control Theory of LLM Prompting (Aman Bhargava, Cameron Witkowski, Manav Shah, Matt Thomson)
https://arxiv.org/abs/2310.04444
LLM Control Theory Seminar (April 2024)
https://www.youtube.com/watch?v=9QtS9sVBFM0
Society for the pursuit of AGI (Cameron founded it)
https://agisociety.mydurable.com/
Roger Federer demo
http://conway.languagegame.io/inference
Neural Cellular Automata, Active Inference, and the Mystery of Biological Computation (Aman)
https://aman-bhargava.com/ai/neuro/neuromorphic/2024/03/25/nca-do-active-inference.html
Aman and Cameron also want to thank Dr. Shi-Zhuo Looi and Prof. Matt Thomson from from Caltech for help and advice on their research. (https://thomsonlab.caltech.edu/ and https://pma.caltech.edu/people/looi-shi-zhuo)
https://x.com/ABhargava2000
https://x.com/witkowski_cam
216集单集
Tất cả các tập
×欢迎使用Player FM
Player FM正在网上搜索高质量的播客,以便您现在享受。它是最好的播客应用程序,适用于安卓、iPhone和网络。注册以跨设备同步订阅。