BBC Radio 5 live’s award winning gaming podcast, discussing the world of video games and games culture.
…
continue reading
Player FM - Internet Radio Done Right
68 subscribers
Checked 20h ago
two 年前已添加!
内容由Tobias Macey提供。所有播客内容(包括剧集、图形和播客描述)均由 Tobias Macey 或其播客平台合作伙伴直接上传和提供。如果您认为有人在未经您许可的情况下使用您的受版权保护的作品,您可以按照此处概述的流程进行操作https://zh.player.fm/legal。
Player FM -播客应用
使用Player FM应用程序离线!
使用Player FM应用程序离线!
值得一听的播客
赞助
M
Mind The Business: Small Business Success Stories


Hitting plateaus is a common milestone in business, but there’s a difference between stability and a rut. In the last installment of this season, we’ll dive into the ways small business owners push beyond plateaus and find new ways to achieve revenue growth. Jannese and Austin wrap up their time in Nashville, Tennessee with a wonderful visit to N.B. Goods to speak with owner Camille Alston . Camille details the times where she hit a wall with profits, the strategies she implemented to increase revenue, what worked, what didn’t, and the important lessons she learned in the process. You won’t want to miss this informative final chapter! Learn more about how QuickBooks can help you grow your business: QuickBooks.com See omnystudio.com/listener for privacy information.…
Enhancing Data Accessibility and Governance with Gravitino
Manage episode 437533509 series 3449056
内容由Tobias Macey提供。所有播客内容(包括剧集、图形和播客描述)均由 Tobias Macey 或其播客平台合作伙伴直接上传和提供。如果您认为有人在未经您许可的情况下使用您的受版权保护的作品,您可以按照此处概述的流程进行操作https://zh.player.fm/legal。
Summary
As data architectures become more elaborate and the number of applications of data increases, it becomes increasingly challenging to locate and access the underlying data. Gravitino was created to provide a single interface to locate and query your data. In this episode Junping Du explains how Gravitino works, the capabilities that it unlocks, and how it fits into your data platform.
Announcements
Parting Question
…
continue reading
As data architectures become more elaborate and the number of applications of data increases, it becomes increasingly challenging to locate and access the underlying data. Gravitino was created to provide a single interface to locate and query your data. In this episode Junping Du explains how Gravitino works, the capabilities that it unlocks, and how it fits into your data platform.
Announcements
- Hello and welcome to the Data Engineering Podcast, the show about modern data management
- Your host is Tobias Macey and today I'm interviewing Junping Du about Gravitino, an open source metadata service for a unified view of all of your schemas
- Introduction
- How did you get involved in the area of data management?
- Can you describe what Gravitino is and the story behind it?
- What problems are you solving with Gravitino?
- What are the methods that teams have relied on in the absence of Gravitino to address those use cases?
- What led to the Hive Metastore being the default for so long?
- What are the opportunities for innovation and new functionality in the metadata service?
- The documentation suggests that Gravitino has overlap with a number of tool categories such as table schema (Hive metastore), metadata repository (Open Metadata), data federation (Trino/Alluxio). What are the capabilities that it can completely replace, and which will require other systems for more comprehensive functionality?
- What are the capabilities that you are explicitly keeping out of scope for Gravitino?
- Can you describe the technical architecture of Gravitino?
- How have the design and scope evolved from when you first started working on it?
- Can you describe how Gravitino integrates into an overall data platform?
- In a typical day, what are the different ways that a data engineer or data analyst might interact with Gravitino?
- One of the features that you highlight is centralized permissions management. Can you describe the access control model that you use for unifying across underlying sources?
- What are the most interesting, innovative, or unexpected ways that you have seen Gravitino used?
- What are the most interesting, unexpected, or challenging lessons that you have learned while working on Gravitino?
- When is Gravitino the wrong choice?
- What do you have planned for the future of Gravitino?
Parting Question
- From your perspective, what is the biggest gap in the tooling or technology for data management today?
- Thank you for listening! Don't forget to check out our other shows. Podcast.__init__ covers the Python language, its community, and the innovative ways it is being used. The AI Engineering Podcast is your guide to the fast-moving world of building AI systems.
- Visit the site to subscribe to the show, sign up for the mailing list, and read the show notes.
- If you've learned something or tried out a project from the show then tell us about it! Email hosts@dataengineeringpodcast.com with your story.
- Gravitino
- Hadoop
- Datastrato
- PyTorch
- Ray
- Data Fabric
- Hive
- Iceberg
- Hive Metastore
- Trino
- OpenMetadata
- Alluxio
- Atlan
- Spark
- Thrift
463集单集
Manage episode 437533509 series 3449056
内容由Tobias Macey提供。所有播客内容(包括剧集、图形和播客描述)均由 Tobias Macey 或其播客平台合作伙伴直接上传和提供。如果您认为有人在未经您许可的情况下使用您的受版权保护的作品,您可以按照此处概述的流程进行操作https://zh.player.fm/legal。
Summary
As data architectures become more elaborate and the number of applications of data increases, it becomes increasingly challenging to locate and access the underlying data. Gravitino was created to provide a single interface to locate and query your data. In this episode Junping Du explains how Gravitino works, the capabilities that it unlocks, and how it fits into your data platform.
Announcements
Parting Question
…
continue reading
As data architectures become more elaborate and the number of applications of data increases, it becomes increasingly challenging to locate and access the underlying data. Gravitino was created to provide a single interface to locate and query your data. In this episode Junping Du explains how Gravitino works, the capabilities that it unlocks, and how it fits into your data platform.
Announcements
- Hello and welcome to the Data Engineering Podcast, the show about modern data management
- Your host is Tobias Macey and today I'm interviewing Junping Du about Gravitino, an open source metadata service for a unified view of all of your schemas
- Introduction
- How did you get involved in the area of data management?
- Can you describe what Gravitino is and the story behind it?
- What problems are you solving with Gravitino?
- What are the methods that teams have relied on in the absence of Gravitino to address those use cases?
- What led to the Hive Metastore being the default for so long?
- What are the opportunities for innovation and new functionality in the metadata service?
- The documentation suggests that Gravitino has overlap with a number of tool categories such as table schema (Hive metastore), metadata repository (Open Metadata), data federation (Trino/Alluxio). What are the capabilities that it can completely replace, and which will require other systems for more comprehensive functionality?
- What are the capabilities that you are explicitly keeping out of scope for Gravitino?
- Can you describe the technical architecture of Gravitino?
- How have the design and scope evolved from when you first started working on it?
- Can you describe how Gravitino integrates into an overall data platform?
- In a typical day, what are the different ways that a data engineer or data analyst might interact with Gravitino?
- One of the features that you highlight is centralized permissions management. Can you describe the access control model that you use for unifying across underlying sources?
- What are the most interesting, innovative, or unexpected ways that you have seen Gravitino used?
- What are the most interesting, unexpected, or challenging lessons that you have learned while working on Gravitino?
- When is Gravitino the wrong choice?
- What do you have planned for the future of Gravitino?
Parting Question
- From your perspective, what is the biggest gap in the tooling or technology for data management today?
- Thank you for listening! Don't forget to check out our other shows. Podcast.__init__ covers the Python language, its community, and the innovative ways it is being used. The AI Engineering Podcast is your guide to the fast-moving world of building AI systems.
- Visit the site to subscribe to the show, sign up for the mailing list, and read the show notes.
- If you've learned something or tried out a project from the show then tell us about it! Email hosts@dataengineeringpodcast.com with your story.
- Gravitino
- Hadoop
- Datastrato
- PyTorch
- Ray
- Data Fabric
- Hive
- Iceberg
- Hive Metastore
- Trino
- OpenMetadata
- Alluxio
- Atlan
- Spark
- Thrift
463集单集
所有剧集
×D
Data Engineering Podcast

Summary In this episode of the Data Engineering Podcast Derek Collison, creator of NATS and CEO of Synadia, talks about the evolution and capabilities of NATS as a multi-paradigm connectivity layer for distributed applications. Derek discusses the challenges and solutions in building distributed systems, and highlights the unique features of NATS that differentiate it from other messaging systems. He delves into the architectural decisions behind NATS, including its ability to handle high-speed global microservices, support for edge computing, and integration with Jetstream for data persistence, and explores the role of NATS in modern data management and its use cases in industries like manufacturing and connected vehicles. Announcements Hello and welcome to the Data Engineering Podcast, the show about modern data management Data migrations are brutal. They drag on for months—sometimes years—burning through resources and crushing team morale. Datafold's AI-powered Migration Agent changes all that. Their unique combination of AI code translation and automated data validation has helped companies complete migrations up to 10 times faster than manual approaches. And they're so confident in their solution, they'll actually guarantee your timeline in writing. Ready to turn your year-long migration into weeks? Visit dataengineeringpodcast.com/datafold today for the details. Your host is Tobias Macey and today I'm interviewing Derek Collison about NATS, a multi-paradigm connectivity layer for distributed applications. Interview Introduction How did you get involved in the area of data management? Can you describe what NATS is and the story behind it? How have your experiences in past roles (cloud foundry, TIBCO messaging systems) informed the core principles of NATS? What other sources of inspiration have you drawn on in the design and evolution of NATS? (e.g. Kafka, RabbitMQ, etc.) There are several patterns and abstractions that NATS can support, many of which overlap with other well-regarded technologies. When designing a system or service, what are the heuristics that should be used to determine whether NATS should act as a replacement or addition to those capabilities? (e.g. considerations of scale, speed, ecosystem compatibility, etc.) There is often a divide in the technologies and architecture used between operational/user-facing applications and data systems. How does the unification of multiple messaging patterns in NATS shift the ways that teams think about the relationship between these use cases? How does the shared communication layer of NATS with multiple protocol and pattern adaptaters reduce the need to replicate data and logic across application and data layers? Can you describe how the core NATS system is architected? How have the design and goals of NATS evolved since you first started working on it? In the time since you first began writing NATS (~2012) there have been several evolutionary stages in both application and data implementation patterns. How have those shifts influenced the direction of the NATS project and its ecosystem? For teams who have an existing architecture, what are some of the patterns for adoption of NATS that allow them to augment or migrate their capabilities? What are some of the ecosystem investments that you and your team have made to ease the adoption and integration of NATS? What are the most interesting, innovative, or unexpected ways that you have seen NATS used? What are the most interesting, unexpected, or challenging lessons that you have learned while working on NATS? When is NATS the wrong choice? What do you have planned for the future of NATS? Contact Info GitHub LinkedIn Parting Question From your perspective, what is the biggest gap in the tooling or technology for data management today? Closing Announcements Thank you for listening! Don't forget to check out our other shows. Podcast.__init__ covers the Python language, its community, and the innovative ways it is being used. The AI Engineering Podcast is your guide to the fast-moving world of building AI systems. Visit the site to subscribe to the show, sign up for the mailing list, and read the show notes. If you've learned something or tried out a project from the show then tell us about it! Email hosts@dataengineeringpodcast.com with your story. Links NATS NATS JetStream Synadia Cloud Foundry TIBCO Applied Physics Lab - Johns Hopkins University Cray Supercomputer RVCM Certified Messaging TIBCO ZMS IBM MQ JMS == Java Message Service RabbitMQ MongoDB NodeJS Redis AMQP == Advanced Message Queueing Protocol Pub/Sub Pattern Circuit Breaker Pattern Zero MQ Akamai Fastly CDN == Content Delivery Network At Most Once At Least Once Exactly Once AWS Kinesis Memcached SQS Segment Rudderstack Podcast Episode DLQ == Dead Letter Queue MQTT == Message Queueing Telemetry Transport NATS Kafka Bridge 10BaseT Network Web Assembly RedPanda Podcast Episode Pulsar Functions mTLS AuthZ (Authorization) AuthN (Authentication) NATS Auth Callouts OPA == Open Policy Agent RAG == Retrieval Augmented Generation AI Engineering Podcast Episode Home Assistant Podcast.__init__ Episode Tailscale Ollama CDC == Change Data Capture gRPC The intro and outro music is from The Hug by The Freak Fandango Orchestra / CC BY-SA…
D
Data Engineering Podcast

Summary In this episode of the Data Engineering Podcast Viktor Kessler, co-founder of Vakmo, talks about the architectural patterns in the lake house enabled by a fast and feature-rich Iceberg catalog. Viktor shares his journey from data warehouses to developing the open-source project, Lakekeeper, an Apache Iceberg REST catalog written in Rust that facilitates building lake houses with essential components like storage, compute, and catalog management. He discusses the importance of metadata in making data actionable, the evolution of data catalogs, and the challenges and innovations in the space, including integration with OpenFGA for fine-grained access control and managing data across formats and compute engines. Announcements Hello and welcome to the Data Engineering Podcast, the show about modern data management Data migrations are brutal. They drag on for months—sometimes years—burning through resources and crushing team morale. Datafold's AI-powered Migration Agent changes all that. Their unique combination of AI code translation and automated data validation has helped companies complete migrations up to 10 times faster than manual approaches. And they're so confident in their solution, they'll actually guarantee your timeline in writing. Ready to turn your year-long migration into weeks? Visit dataengineeringpodcast.com/datafold today for the details. Your host is Tobias Macey and today I'm interviewing Viktor Kessler about architectural patterns in the lakehouse that are unlocked by a fast and feature-rich Iceberg catalog Interview Introduction How did you get involved in the area of data management? Can you describe what LakeKeeper is and the story behind it? What is the core of the problem that you are addressing? There has been a lot of activity in the catalog space recently. What are the driving forces that have highlighted the need for a better metadata catalog in the data lake/distributed data ecosystem? How would you characterize the feature sets/problem spaces that different entrants are focused on addressing? Iceberg as a table format has gained a lot of attention and adoption across the data ecosystem. The REST catalog format has opened the door for numerous implementations. What are the opportunities for innovation and improving user experience in that space? What is the role of the catalog in managing security and governance? (AuthZ, auditing, etc.) What are the channels for propagating identity and permissions to compute engines? (how do you avoid head-scratching about permission denied situations) Can you describe how LakeKeeper is implemented? How have the design and goals of the project changed since you first started working on it? For someone who has an existing set of Iceberg tables and catalog, what does the migration process look like? What new workflows or capabilities does LakeKeeper enable for data teams using Iceberg tables across one or more compute frameworks? What are the most interesting, innovative, or unexpected ways that you have seen LakeKeeper used? What are the most interesting, unexpected, or challenging lessons that you have learned while working on LakeKeeper? When is LakeKeeper the wrong choice? What do you have planned for the future of LakeKeeper? Contact Info LinkedIn Parting Question From your perspective, what is the biggest gap in the tooling or technology for data management today? Closing Announcements Thank you for listening! Don't forget to check out our other shows. Podcast.__init__ covers the Python language, its community, and the innovative ways it is being used. The AI Engineering Podcast is your guide to the fast-moving world of building AI systems. Visit the site to subscribe to the show, sign up for the mailing list, and read the show notes. If you've learned something or tried out a project from the show then tell us about it! Email hosts@dataengineeringpodcast.com with your story. Links LakeKeeper SAP Microsoft Access Microsoft Excel Apache Iceberg Podcast Episode Iceberg REST Catalog PyIceberg Spark Trino Dremio Hive Metastore Hadoop NATS Polars DuckDB Podcast Episode DataFusion Atlan Podcast Episode Open Metadata Podcast Episode Apache Atlas OpenFGA Hudi Podcast Episode Delta Lake Podcast Episode Lance Table Format Podcast Episode Unity Catalog Polaris Catalog Apache Gravitino Podcast Episode Keycloak Open Policy Agent (OPA) Apache Ranger Apache NiFi The intro and outro music is from The Hug by The Freak Fandango Orchestra / CC BY-SA…
D
Data Engineering Podcast

Summary In this episode of the Data Engineering Podcast Jeremy Edberg, CEO of DBOS, about durable execution and its impact on designing and implementing business logic for data systems. Jeremy explains how DBOS's serverless platform and orchestrator provide local resilience and reduce operational overhead, ensuring exactly-once execution in distributed systems through the use of the Transact library. He discusses the importance of version management in long-running workflows and how DBOS simplifies system design by reducing infrastructure needs like queues and CI pipelines, making it beneficial for data pipelines, AI workloads, and agentic AI. Announcements Hello and welcome to the Data Engineering Podcast, the show about modern data management Data migrations are brutal. They drag on for months—sometimes years—burning through resources and crushing team morale. Datafold's AI-powered Migration Agent changes all that. Their unique combination of AI code translation and automated data validation has helped companies complete migrations up to 10 times faster than manual approaches. And they're so confident in their solution, they'll actually guarantee your timeline in writing. Ready to turn your year-long migration into weeks? Visit dataengineeringpodcast.com/datafold today for the details. Your host is Tobias Macey and today I'm interviewing Jeremy Edberg about durable execution and how it influences the design and implementation of business logic Interview Introduction How did you get involved in the area of data management? Can you describe what DBOS is and the story behind it? What is durable execution? What are some of the notable ways that inclusion of durable execution in an application architecture changes the ways that the rest of the application is implemented? (e.g. error handling, logic flow, etc.) Many data pipelines involve complex, multi-step workflows. How does DBOS simplify the creation and management of resilient data pipelines? How does durable execution impact the operational complexity of data management systems? One of the complexities in durable execution is managing code/data changes to workflows while existing executions are still processing. What are some of the useful patterns for addressing that challenge and how does DBOS help? Can you describe how DBOS is architected? How have the design and goals of the system changed since you first started working on it? What are the characteristics of Postgres that make it suitable for the persistence mechanism of DBOS? What are the guiding principles that you rely on to determine the boundaries between the open source and commercial elements of DBOS? What are the most interesting, innovative, or unexpected ways that you have seen DBOS used? What are the most interesting, unexpected, or challenging lessons that you have learned while working on DBOS? When is DBOS the wrong choice? What do you have planned for the future of DBOS? Contact Info LinkedIn Parting Question From your perspective, what is the biggest gap in the tooling or technology for data management today? Closing Announcements Thank you for listening! Don't forget to check out our other shows. Podcast.__init__ covers the Python language, its community, and the innovative ways it is being used. The AI Engineering Podcast is your guide to the fast-moving world of building AI systems. Visit the site to subscribe to the show, sign up for the mailing list, and read the show notes. If you've learned something or tried out a project from the show then tell us about it! Email hosts@dataengineeringpodcast.com with your story. Links DBOS Exactly Once Semantics Temporal Sempahore Postgres DBOS Transact Python Typescript Idempotency Keys Agentic AI State Machine YugabyteDB Podcast Episode CockroachDB Supabase Neon Podcast Episode Airflow The intro and outro music is from The Hug by The Freak Fandango Orchestra / CC BY-SA…
D
Data Engineering Podcast

Summary In this episode of the Data Engineering Podcast Roman Gershman, CTO and founder of Dragonfly DB, explores the development and impact of high-speed in-memory databases. Roman shares his experience creating a more efficient alternative to Redis, focusing on performance gains, scalability, and cost efficiency, while addressing limitations such as high throughput and low latency scenarios. He explains how Dragonfly DB solves operational complexities for users and delves into its technical aspects, including maintaining compatibility with Redis while innovating on memory efficiency. Roman discusses the importance of cost efficiency and operational simplicity in driving adoption and shares insights on the broader ecosystem of in-memory data stores, future directions like SSD tiering and vector search capabilities, and the lessons learned from building a new database engine. Announcements Hello and welcome to the Data Engineering Podcast, the show about modern data management Data migrations are brutal. They drag on for months—sometimes years—burning through resources and crushing team morale. Datafold's AI-powered Migration Agent changes all that. Their unique combination of AI code translation and automated data validation has helped companies complete migrations up to 10 times faster than manual approaches. And they're so confident in their solution, they'll actually guarantee your timeline in writing. Ready to turn your year-long migration into weeks? Visit dataengineeringpodcast.com/datafold today for the details. Your host is Tobias Macey and today I'm interviewing Roman Gershman about building a high-speed in-memory database and the impact of the performance gains on data applications Interview Introduction How did you get involved in the area of data management? Can you describe what DragonflyDB is and the story behind it? What is the core problem/use case that is solved by making a "faster Redis"? The other major player in the high performance key/value database space is Aerospike. What are the heuristics that an engineer should use to determine whether to use that vs. Dragonfly/Redis? Common use cases for Redis involve application caches and queueing (e.g. Celery/RQ). What are some of the other applications that you have seen Redis/Dragonfly used for, particularly in data engineering use cases? There is a piece of tribal wisdom that it takes 10 years for a database to iron out all of the kinks. At the same time, there have been substantial investments in commoditizing the underlying components of database engines. Can you describe how you approached the implementation of DragonflyDB to arive at a functional and reliable implementation? What are the architectural elements that contribute to the performance and scalability benefits of Dragonfly? How have the design and goals of the system changed since you first started working on it? For teams who migrate from Redis to Dragonfly, beyond the cost savings what are some of the ways that it changes the ways that they think about their overall system design? What are the most interesting, innovative, or unexpected ways that you have seen Dragonfly used? What are the most interesting, unexpected, or challenging lessons that you have learned while working on DragonflyDB? When is DragonflyDB the wrong choice? What do you have planned for the future of DragonflyDB? Contact Info GitHub LinkedIn Parting Question From your perspective, what is the biggest gap in the tooling or technology for data management today? Closing Announcements Thank you for listening! Don't forget to check out our other shows. Podcast.__init__ covers the Python language, its community, and the innovative ways it is being used. The AI Engineering Podcast is your guide to the fast-moving world of building AI systems. Visit the site to subscribe to the show, sign up for the mailing list, and read the show notes. If you've learned something or tried out a project from the show then tell us about it! Email hosts@dataengineeringpodcast.com with your story. Links DragonflyDB Redis Elasticache ValKey Aerospike Laravel Sidekiq Celery Seastar Framework Shared-Nothing Architecture io_uring midi-redis Dunning-Kruger Effect Rust The intro and outro music is from The Hug by The Freak Fandango Orchestra / CC BY-SA…
Summary In this episode of the Data Engineering Podcast Sean Knapp, CEO of Ascend.io, explores the intersection of AI and data engineering. He discusses the evolution of data engineering and the role of AI in automating processes, alleviating burdens on data engineers, and enabling them to focus on complex tasks and innovation. The conversation covers the challenges and opportunities presented by AI, including the need for intelligent tooling and its potential to streamline data engineering processes. Sean and Tobias also delve into the impact of generative AI on data engineering, highlighting its ability to accelerate development, improve governance, and enhance productivity, while also noting the current limitations and future potential of AI in the field. Announcements Hello and welcome to the Data Engineering Podcast, the show about modern data management Data migrations are brutal. They drag on for months—sometimes years—burning through resources and crushing team morale. Datafold's AI-powered Migration Agent changes all that. Their unique combination of AI code translation and automated data validation has helped companies complete migrations up to 10 times faster than manual approaches. And they're so confident in their solution, they'll actually guarantee your timeline in writing. Ready to turn your year-long migration into weeks? Visit dataengineeringpodcast.com/datafold today for the details. Your host is Tobias Macey and today I'm interviewing Sean Knapp about how Ascend is incorporating AI into their platform to help you keep up with the rapid rate of change Interview Introduction How did you get involved in the area of data management? Can you describe what Ascend is and the story behind it? The last time we spoke was August of 2022 . What are the most notable or interesting evolutions in your platform since then? In that same time "AI" has taken up all of the oxygen in the data ecosystem. How has that impacted the ways that you and your customers think about their priorities? The introduction of AI as an API has caused many organizations to try and leap-frog their data maturity journey and jump straight to building with advanced capabilities. How is that impacting the pressures and priorities felt by data teams? At the same time that AI-focused product goals are straining data teams capacities, AI also has the potential to act as an accelerator to their work. What are the roadblocks/speedbumps that are in the way of that capability? Many data teams are incorporating AI tools into parts of their workflow, but it can be clunky and cumbersome. How are you thinking about the fundamental changes in how your platform works with AI at its center? Can you describe the technical architecture that you have evolved toward that allows for AI to drive the experience rather than being a bolt-on? What are the concrete impacts that these new capabilities have on teams who are using Ascend? What are the most interesting, innovative, or unexpected ways that you have seen Ascend + AI used? What are the most interesting, unexpected, or challenging lessons that you have learned while working on incorporating AI into the core of Ascend? When is Ascend the wrong choice? What do you have planned for the future of AI in Ascend? Contact Info LinkedIn Parting Question From your perspective, what is the biggest gap in the tooling or technology for data management today? Closing Announcements Thank you for listening! Don't forget to check out our other shows. Podcast.__init__ covers the Python language, its community, and the innovative ways it is being used. The AI Engineering Podcast is your guide to the fast-moving world of building AI systems. Visit the site to subscribe to the show, sign up for the mailing list, and read the show notes. If you've learned something or tried out a project from the show then tell us about it! Email hosts@dataengineeringpodcast.com with your story. Links Ascend Cursor AI Code Editor Devin GitHub Copilot OpenAI DeepResearch S3 Tables AWS Glue AWS Bedrock Snowpark Co-Intelligence : Living and Working with AI by Ethan Mollick (affiliate link) OpenAI o3 The intro and outro music is from The Hug by The Freak Fandango Orchestra / CC BY-SA…
D
Data Engineering Podcast

Summary In this episode of the Data Engineering Podcast Pete DeJoy, co-founder and product lead at Astronomer, talks about building and managing Airflow pipelines on Astronomer and the upcoming improvements in Airflow 3. Pete shares his journey into data engineering, discusses Astronomer's contributions to the Airflow project, and highlights the critical role of Airflow in powering operational data products. He covers the evolution of Airflow, its position in the data ecosystem, and the challenges faced by data engineers, including infrastructure management and observability. The conversation also touches on the upcoming Airflow 3 release, which introduces data awareness, architectural improvements, and multi-language support, and Astronomer's observability suite, Astro Observe, which provides insights and proactive recommendations for Airflow users. Announcements Hello and welcome to the Data Engineering Podcast, the show about modern data management Data migrations are brutal. They drag on for months—sometimes years—burning through resources and crushing team morale. Datafold's AI-powered Migration Agent changes all that. Their unique combination of AI code translation and automated data validation has helped companies complete migrations up to 10 times faster than manual approaches. And they're so confident in their solution, they'll actually guarantee your timeline in writing. Ready to turn your year-long migration into weeks? Visit dataengineeringpodcast.com/datafold today for the details. Your host is Tobias Macey and today I'm interviewing Pete DeJoy about building and managing Airflow pipelines on Astronomer and the upcoming improvements in Airflow 3 Interview Introduction Can you describe what Astronomer is and the story behind it? How would you characterize the relationship between Airflow and Astronomer? Astronomer just released your State of Airflow 2025 Report yesterday and it is the largest data engineering survey ever with over 5,000 respondents. Can you talk a bit about top level findings in the report? What about the overall growth of the Airflow project over time? How have the focus and features of Astronomer changed since it was last featured on the show in 2017? Astro Observe GA’d in early February, what does the addition of pipeline observability mean for your customers? What are other capabilities similar in scope to observability that Astronomer is looking at adding to the platform? Why is Airflow so critical in providing an elevated Observability–or cataloging, or something simlar - experience in a DataOps platform? What are the notable evolutions in the Airflow project and ecosystem in that time? What are the core improvements that are planned for Airflow 3.0? What are the most interesting, innovative, or unexpected ways that you have seen Astro used? What are the most interesting, unexpected, or challenging lessons that you have learned while working on Airflow and Astro? What do you have planned for the future of Astro/Astronomer/Airflow? Contact Info LinkedIn Parting Question From your perspective, what is the biggest gap in the tooling or technology for data management today? Closing Announcements Thank you for listening! Don't forget to check out our other shows. Podcast.__init__ covers the Python language, its community, and the innovative ways it is being used. The AI Engineering Podcast is your guide to the fast-moving world of building AI systems. Visit the site to subscribe to the show, sign up for the mailing list, and read the show notes. If you've learned something or tried out a project from the show then tell us about it! Email hosts@dataengineeringpodcast.com with your story. Links Astronomer Airflow Maxime Beauchemin MongoDB Databricks Confluent Spark Kafka Dagster Podcast Episode Prefect Airflow 3 The Rise of the Data Engineer blog post dbt Jupyter Notebook Zapier cosmos library for dbt in Airflow Ruff Airflow Custom Operator Snowflake The intro and outro music is from The Hug by The Freak Fandango Orchestra / CC BY-SA…
D
Data Engineering Podcast

Summary In this episode of the Data Engineering Podcast Rajan Goyal, CEO and co-founder of Datapelago, talks about improving efficiencies in data processing by reimagining system architecture. Rajan explains the shift from hyperconverged to disaggregated and composable infrastructure, highlighting the importance of accelerated computing in modern data centers. He discusses the evolution from proprietary to open, composable stacks, emphasizing the role of open table formats and the need for a universal data processing engine, and outlines Datapelago's strategy to leverage existing frameworks like Spark and Trino while providing accelerated computing benefits. Announcements Hello and welcome to the Data Engineering Podcast, the show about modern data management Data migrations are brutal. They drag on for months—sometimes years—burning through resources and crushing team morale. Datafold's AI-powered Migration Agent changes all that. Their unique combination of AI code translation and automated data validation has helped companies complete migrations up to 10 times faster than manual approaches. And they're so confident in their solution, they'll actually guarantee your timeline in writing. Ready to turn your year-long migration into weeks? Visit dataengineeringpodcast.com/datafold today for the details. Your host is Tobias Macey and today I'm interviewing Rajan Goyal about how to drastically improve efficiencies in data processing by re-imagining the system architecture Interview Introduction How did you get involved in the area of data management? Can you start by outlining the main factors that contribute to performance challenges in data lake environments? The different components of open data processing systems have evolved from different starting points with different objectives. In your experience, how has that un-planned and un-synchronized evolution of the ecosystem hindered the capabilities and adoption of open technologies? The introduction of a new cross-cutting capability (e.g. Iceberg) has typically taken a substantial amount of time to gain support across different engines and ecosystems. What do you see as the point of highest leverage to improve the capabilities of the entire stack with the least amount of co-ordination? What was the motivating insight that led you to invest in the technology that powers Datapelago? Can you describe the system design of Datapelago and how it integrates with existing data engines? The growth in the generation and application of unstructured data is a notable shift in the work being done by data teams. What are the areas of overlap in the fundamental nature of data (whether structured, semi-structured, or unstructured) that you are able to exploit to bridge the processing gap? What are the most interesting, innovative, or unexpected ways that you have seen Datapelago used? What are the most interesting, unexpected, or challenging lessons that you have learned while working on Datapelago? When is Datapelago the wrong choice? What do you have planned for the future of Datapelago? Contact Info LinkedIn Parting Question From your perspective, what is the biggest gap in the tooling or technology for data management today? Links Datapelago MIPS Architecture ARM Architecture AWS Nitro Mellanox Nvidia Von Neumann Architecture TPU == Tensor Processing Unit FPGA == Field-Programmable Gate Array Spark Trino Iceberg Podcast Episode Delta Lake Podcast Episode Hudi Podcast Episode Apache Gluten Intermediate Representation Turing Completeness LLVM Amdahl's Law LSTM == Long Short-Term Memory The intro and outro music is from The Hug by The Freak Fandango Orchestra / CC BY-SA…
D
Data Engineering Podcast

Summary In this episode of the Data Engineering Podcast Gleb Mezhanskiy, CEO and co-founder of DataFold, talks about the intersection of AI and data engineering. He discusses the challenges and opportunities of integrating AI into data engineering, particularly using large language models (LLMs) to enhance productivity and reduce manual toil. The conversation covers the potential of AI to transform data engineering tasks, such as text-to-SQL interfaces and creating semantic graphs to improve data accessibility, and explores practical applications of LLMs in automating code reviews, testing, and understanding data lineage. Announcements Hello and welcome to the Data Engineering Podcast, the show about modern data management Data migrations are brutal. They drag on for months—sometimes years—burning through resources and crushing team morale. Datafold's AI-powered Migration Agent changes all that. Their unique combination of AI code translation and automated data validation has helped companies complete migrations up to 10 times faster than manual approaches. And they're so confident in their solution, they'll actually guarantee your timeline in writing. Ready to turn your year-long migration into weeks? Visit dataengineeringpodcast.com/datafold today for the details. Your host is Tobias Macey and today I'm interviewing Gleb Mezhanskiy about Interview Introduction How did you get involved in the area of data management? modern data stack is dead where is AI in the data stack? "buy our tool to ship AI" opportunities for LLM in DE workflow Contact Info LinkedIn Parting Question From your perspective, what is the biggest gap in the tooling or technology for data management today? Closing Announcements Thank you for listening! Don't forget to check out our other shows. Podcast.__init__ covers the Python language, its community, and the innovative ways it is being used. The AI Engineering Podcast is your guide to the fast-moving world of building AI systems. Visit the site to subscribe to the show, sign up for the mailing list, and read the show notes. If you've learned something or tried out a project from the show then tell us about it! Email hosts@dataengineeringpodcast.com with your story. Links Datafold Copilot Cursor IDE AI Agents DataChat AI Engineering Podcast Episode Metrics Layer Emacs LangChain LangGraph CrewAI The intro and outro music is from The Hug by The Freak Fandango Orchestra / CC BY-SA…
D
Data Engineering Podcast

Summary In this episode of the Data Engineering Podcast Bartosz Mikulski talks about preparing data for AI applications. Bartosz shares his journey from data engineering to MLOps and emphasizes the importance of data testing over software development in AI contexts. He discusses the types of data assets required for AI applications, including extensive test datasets, especially in generative AI, and explains the differences in data requirements for various AI application styles. The conversation also explores the skills data engineers need to transition into AI, such as familiarity with vector databases and new data modeling strategies, and highlights the challenges of evolving AI applications, including frequent reprocessing of data when changing chunking strategies or embedding models. Announcements Hello and welcome to the Data Engineering Podcast, the show about modern data management Data migrations are brutal. They drag on for months—sometimes years—burning through resources and crushing team morale. Datafold's AI-powered Migration Agent changes all that. Their unique combination of AI code translation and automated data validation has helped companies complete migrations up to 10 times faster than manual approaches. And they're so confident in their solution, they'll actually guarantee your timeline in writing. Ready to turn your year-long migration into weeks? Visit dataengineeringpodcast.com/datafold today for the details. Your host is Tobias Macey and today I'm interviewing Bartosz Mikulski about how to prepare data for use in AI applications Interview Introduction How did you get involved in the area of data management? Can you start by outlining some of the main categories of data assets that are needed for AI applications? How does the nature of the application change those requirements? (e.g. RAG app vs. agent, etc.) How do the different assets map to the stages of the application lifecycle? What are some of the common roles and divisions of responsibility that you see in the construction and operation of a "typical" AI application? For data engineers who are used to data warehousing/BI, what are the skills that map to AI apps? What are some of the data modeling patterns that are needed to support AI apps? chunking strategies metadata management What are the new categories of data that data engineers need to manage in the context of AI applications? agent memory generation/evolution conversation history management data collection for fine tuning What are some of the notable evolutions in the space of AI applications and their patterns that have happened in the past ~1-2 years that relate to the responsibilities of data engineers? What are some of the skills gaps that teams should be aware of and identify training opportunities for? What are the most interesting, innovative, or unexpected ways that you have seen data teams address the needs of AI applications? What are the most interesting, unexpected, or challenging lessons that you have learned while working on AI applications and their reliance on data? What are some of the emerging trends that you are paying particular attention to? Contact Info Website LinkedIn Parting Question From your perspective, what is the biggest gap in the tooling or technology for data management today? Closing Announcements Thank you for listening! Don't forget to check out our other shows. Podcast.__init__ covers the Python language, its community, and the innovative ways it is being used. The AI Engineering Podcast is your guide to the fast-moving world of building AI systems. Visit the site to subscribe to the show, sign up for the mailing list, and read the show notes. If you've learned something or tried out a project from the show then tell us about it! Email hosts@dataengineeringpodcast.com with your story. Links Spark Ray Chunking Strategies Hypothetical document embeddings Model Fine Tuning Prompt Compression The intro and outro music is from The Hug by The Freak Fandango Orchestra / CC BY-SA…
D
Data Engineering Podcast

Summary In this episode of the Data Engineering Podcast Andrew Luo, CEO of OneSchema, talks about handling CSV data in business operations. Andrew shares his background in data engineering and CRM migration, which led to the creation of OneSchema, a platform designed to automate CSV imports and improve data validation processes. He discusses the challenges of working with CSVs, including inconsistent type representation, lack of schema information, and technical complexities, and explains how OneSchema addresses these issues using multiple CSV parsers and AI for data type inference and validation. Andrew highlights the business case for OneSchema, emphasizing efficiency gains for companies dealing with large volumes of CSV data, and shares plans to expand support for other data formats and integrate AI-driven transformation packs for specific industries. Announcements Hello and welcome to the Data Engineering Podcast, the show about modern data management Data migrations are brutal. They drag on for months—sometimes years—burning through resources and crushing team morale. Datafold's AI-powered Migration Agent changes all that. Their unique combination of AI code translation and automated data validation has helped companies complete migrations up to 10 times faster than manual approaches. And they're so confident in their solution, they'll actually guarantee your timeline in writing. Ready to turn your year-long migration into weeks? Visit dataengineeringpodcast.com/datafold today for the details. Your host is Tobias Macey and today I'm interviewing Andrew Luo about how OneSchema addresses the headaches of dealing with CSV data for your business Interview Introduction How did you get involved in the area of data management? Despite the years of evolution and improvement in data storage and interchange formats, CSVs are just as prevalent as ever. What are your opinions/theories on why they are so ubiquitous? What are some of the major sources of CSV data for teams that rely on them for business and analytical processes? The most obvious challenge with CSVs is their lack of type information, but they are notorious for having numerous other problems. What are some of the other major challenges involved with using CSVs for data interchange/ingestion? Can you describe what you are building at OneSchema and the story behind it? What are the core problems that you are solving, and for whom? Can you describe how you have architected your platform to be able to manage the variety, volume, and multi-tenancy of data that you process? How have the design and goals of the product changed since you first started working on it? What are some of the major performance issues that you have encountered while dealing with CSV data at scale? What are some of the most surprising things that you have learned about CSVs in the process of building OneSchema? What are the most interesting, innovative, or unexpected ways that you have seen OneSchema used? What are the most interesting, unexpected, or challenging lessons that you have learned while working on OneSchema? When is OneSchema the wrong choice? What do you have planned for the future of OneSchema? Contact Info LinkedIn Parting Question From your perspective, what is the biggest gap in the tooling or technology for data management today? Closing Announcements Thank you for listening! Don't forget to check out our other shows. Podcast.__init__ covers the Python language, its community, and the innovative ways it is being used. The AI Engineering Podcast is your guide to the fast-moving world of building AI systems. Visit the site to subscribe to the show, sign up for the mailing list, and read the show notes. If you've learned something or tried out a project from the show then tell us about it! Email hosts@dataengineeringpodcast.com with your story. Links OneSchema EDI == Electronic Data Interchange UTF-8 BOM (Byte Order Mark) Characters SOAP CSV RFC Iceberg SSIS == SQL Server Integration Services MS Access Datafusion JSON Schema SFTP == Secure File Transfer Protocol The intro and outro music is from The Hug by The Freak Fandango Orchestra / CC BY-SA…
D
Data Engineering Podcast

Summary In this episode of the Data Engineering Podcast Dan Bruckner, co-founder and CTO of Tamr, talks about the application of machine learning (ML) and artificial intelligence (AI) in master data management (MDM). Dan shares his journey from working at CERN to becoming a data expert and discusses the challenges of reconciling large-scale organizational data. He explains how data silos arise from independent teams and highlights the importance of combining traditional techniques with modern AI to address the nuances of data reconciliation. Dan emphasizes the transformative potential of large language models (LLMs) in creating more natural user experiences, improving trust in AI-driven data solutions, and simplifying complex data management processes. He also discusses the balance between using AI for complex data problems and the necessity of human oversight to ensure accuracy and trust. Announcements Hello and welcome to the Data Engineering Podcast, the show about modern data management Data migrations are brutal. They drag on for months—sometimes years—burning through resources and crushing team morale. Datafold's AI-powered Migration Agent changes all that. Their unique combination of AI code translation and automated data validation has helped companies complete migrations up to 10 times faster than manual approaches. And they're so confident in their solution, they'll actually guarantee your timeline in writing. Ready to turn your year-long migration into weeks? Visit dataengineeringpodcast.com/datafold today for the details. As a listener of the Data Engineering Podcast you clearly care about data and how it affects your organization and the world. For even more perspective on the ways that data impacts everything around us don't miss Data Citizens® Dialogues, the forward-thinking podcast brought to you by Collibra. You'll get further insights from industry leaders, innovators, and executives in the world's largest companies on the topics that are top of mind for everyone. In every episode of Data Citizens® Dialogues, industry leaders unpack data’s impact on the world; like in their episode “The Secret Sauce Behind McDonald’s Data Strategy”, which digs into how AI-driven tools can be used to support crew efficiency and customer interactions. In particular I appreciate the ability to hear about the challenges that enterprise scale businesses are tackling in this fast-moving field. The Data Citizens Dialogues podcast is bringing the data conversation to you, so start listening now! Follow Data Citizens Dialogues on Apple, Spotify, YouTube, or wherever you get your podcasts. Your host is Tobias Macey and today I'm interviewing Dan Bruckner about the application of ML and AI techniques to the challenge of reconciling data at the scale of business Interview Introduction How did you get involved in the area of data management? Can you start by giving an overview of the different ways that organizational data becomes unwieldy and needs to be consolidated and reconciled? How does that reconciliation relate to the practice of "master data management" What are the scaling challenges with the current set of practices for reconciling data? ML has been applied to data cleaning for a long time in the form of entity resolution, etc. How has the landscape evolved or matured in recent years? What (if any) transformative capabilities do LLMs introduce? What are the missing pieces/improvements that are necessary to make current AI systems usable out-of-the-box for data cleaning? What are the strategic decisions that need to be addressed when implementing ML/AI techniques in the data cleaning/reconciliation process? What are the risks involved in bringing ML to bear on data cleaning for inexperienced teams? What are the most interesting, innovative, or unexpected ways that you have seen ML techniques used in data resolution? What are the most interesting, unexpected, or challenging lessons that you have learned while working on using ML/AI in master data management? When is ML/AI the wrong choice for data cleaning/reconciliation? What are your hopes/predictions for the future of ML/AI applications in MDM and data cleaning? Contact Info LinkedIn Parting Question From your perspective, what is the biggest gap in the tooling or technology for data management today? Closing Announcements Thank you for listening! Don't forget to check out our other shows. Podcast.__init__ covers the Python language, its community, and the innovative ways it is being used. The AI Engineering Podcast is your guide to the fast-moving world of building AI systems. Visit the site to subscribe to the show, sign up for the mailing list, and read the show notes. If you've learned something or tried out a project from the show then tell us about it! Email hosts@dataengineeringpodcast.com with your story. Links Tamr Master Data Management CERN LHC Michael Stonebraker Conway's Law Expert Systems Information Retrieval Active Learning The intro and outro music is from The Hug by The Freak Fandango Orchestra / CC BY-SA…
D
Data Engineering Podcast

Summary In this episode of the Data Engineering Podcast Lior Barak shares his insights on developing a three-year strategic vision for data management. He discusses the importance of having a strategic plan for data, highlighting the need for data teams to focus on impact rather than just enablement. He introduces the concept of a "data vision board" and explains how it can help organizations outline their strategic vision by considering three key forces: regulation, stakeholders, and organizational goals. Lior emphasizes the importance of balancing short-term pressures with long-term strategic goals, quantifying the cost of data issues to prioritize effectively, and maintaining the strategic vision as a living document through regular reviews. He encourages data teams to shift from being enablers to impact creators and provides practical advice on implementing a data vision board, setting clear KPIs, and embracing a product mindset to create tangible business impacts through strategic data management. Announcements Hello and welcome to the Data Engineering Podcast, the show about modern data management It’s 2024, why are we still doing data migrations by hand? Teams spend months—sometimes years—manually converting queries and validating data, burning resources and crushing morale. Datafold's AI-powered Migration Agent brings migrations into the modern era. Their unique combination of AI code translation and automated data validation has helped companies complete migrations up to 10 times faster than manual approaches. And they're so confident in their solution, they'll actually guarantee your timeline in writing. Ready to turn your year-long migration into weeks? Visit dataengineeringpodcast.com/datafold today to learn how Datafold can automate your migration and ensure source to target parity. Your host is Tobias Macey and today I'm interviewing Lior Barak about how to develop your three year strategic vision for data Interview Introduction How did you get involved in the area of data management? Can you start by giving an outline of the types of problems that occur as a result of not developing a strategic plan for an organization's data systems? What is the format that you recommend for capturing that strategic vision? What are the types of decisions and details that you believe should be included in a vision statement? Why is a 3 year horizon beneficial? What does that scale of time encourage/discourage in the debate and decision-making process? Who are the personas that should be included in the process of developing this strategy document? Can you walk us through the steps and processes involved in developing the data vision board for an organization? What are the time-frames or milestones that should lead to revisiting and revising the strategic objectives? What are the most interesting, innovative, or unexpected ways that you have seen a data vision strategy used? What are the most interesting, unexpected, or challenging lessons that you have learned while working on data strategy development? When is a data vision board the wrong choice? What are some additional resources or practices that you recommend teams invest in as a supplement to this strategic vision exercise? Contact Info LinkedIn Substack Parting Question From your perspective, what is the biggest gap in the tooling or technology for data management today? Closing Announcements Thank you for listening! Don't forget to check out our other shows. Podcast.__init__ covers the Python language, its community, and the innovative ways it is being used. The AI Engineering Podcast is your guide to the fast-moving world of building AI systems. Visit the site to subscribe to the show, sign up for the mailing list, and read the show notes. If you've learned something or tried out a project from the show then tell us about it! Email hosts@dataengineeringpodcast.com with your story. Links Vision Board Overview Episode 397: Defining A Strategy For Your Data Products Minto Pyramid Principle KPI == Key Performance Indicator OKR == Objectives and Key Results Phil Jackson: Eleven Rings (affiliate link) The intro and outro music is from The Hug by The Freak Fandango Orchestra / CC BY-SA…
D
Data Engineering Podcast

Summary The core task of data engineering is managing the flows of data through an organization. In order to ensure those flows are executing on schedule and without error is the role of the data orchestrator. Which orchestration engine you choose impacts the ways that you architect the rest of your data platform. In this episode Hugo Lu shares his thoughts as the founder of an orchestration company on how to think about data orchestration and data platform design as we navigate the current era of data engineering. Announcements Hello and welcome to the Data Engineering Podcast, the show about modern data management It’s 2024, why are we still doing data migrations by hand? Teams spend months—sometimes years—manually converting queries and validating data, burning resources and crushing morale. Datafold's AI-powered Migration Agent brings migrations into the modern era. Their unique combination of AI code translation and automated data validation has helped companies complete migrations up to 10 times faster than manual approaches. And they're so confident in their solution, they'll actually guarantee your timeline in writing. Ready to turn your year-long migration into weeks? Visit dataengineeringpodcast.com/datafold today to learn how Datafold can automate your migration and ensure source to target parity. As a listener of the Data Engineering Podcast you clearly care about data and how it affects your organization and the world. For even more perspective on the ways that data impacts everything around us don't miss Data Citizens® Dialogues, the forward-thinking podcast brought to you by Collibra. You'll get further insights from industry leaders, innovators, and executives in the world's largest companies on the topics that are top of mind for everyone. In every episode of Data Citizens® Dialogues, industry leaders unpack data’s impact on the world, from big picture questions like AI governance and data sharing to more nuanced questions like, how do we balance offense and defense in data management? In particular I appreciate the ability to hear about the challenges that enterprise scale businesses are tackling in this fast-moving field. The Data Citizens Dialogues podcast is bringing the data conversation to you, so start listening now! Follow Data Citizens Dialogues on Apple, Spotify, YouTube, or wherever you get your podcasts. Your host is Tobias Macey and today I'm interviewing Hugo Lu about the data platform and orchestration ecosystem and how to navigate the available options Interview Introduction How did you get involved in building data platforms? Can you describe what an orchestrator is in the context of data platforms? There are many other contexts in which orchestration is necessary. What are some examples of how orchestrators have adapted (or failed to adapt) to the times? What are the core features that are necessary for an orchestrator to have when dealing with data-oriented workflows? Beyond the bare necessities, what are some of the other features and design considerations that go into building a first-class dat platform or orchestration system? There have been several generations of orchestration engines over the past several years. How would you characterize the different coarse groupings of orchestration engines across those generational boundaries? How do the characteristics of a data orchestrator influence the overarching architecture of an organization's data platform/data operations? What about the reverse? How have the cycles of ML and AI workflow requirements impacted the design requirements for data orchestrators? What are the most interesting, innovative, or unexpected ways that you have seen data orchestrators used? What are the most interesting, unexpected, or challenging lessons that you have learned while working on data orchestration? When is an orchestrator the wrong choice? What are your predictions and/or hopes for the future of data orchestration? Contact Info Medium LinkedIn Parting Question From your perspective, what is the biggest thing data teams are missing in the technology today? Closing Announcements Thank you for listening! Don't forget to check out our other shows. Podcast.__init__ covers the Python language, its community, and the innovative ways it is being used. The AI Engineering Podcast is your guide to the fast-moving world of building AI systems. Visit the site to subscribe to the show, sign up for the mailing list, and read the show notes. If you've learned something or tried out a project from the show then tell us about it! Email hosts@dataengineeringpodcast.com with your story. Links Orchestra Previous Episode: Overview Of The State Of Data Orchestration Cron ArgoCD DAG Kubernetes Data Mesh Airflow SSIS == SQL Server Integration Services Pentaho Kettle DataVolo NiFi Podcast Episode Dagster gRPC Coalesce Podcast Episode dbt DataHub Palantir The intro and outro music is from The Hug by The Freak Fandango Orchestra / CC BY-SA…
D
Data Engineering Podcast

Summary In this episode of the Data Engineering Podcast the inimitable Max Beauchemin talks about reusability in data pipelines. The conversation explores the "write everything twice" problem, where similar pipelines are built without code reuse, and discusses the challenges of managing different SQL dialects and relational databases. Max also touches on the evolving role of data engineers, drawing parallels with front-end engineering, and suggests that generative AI could facilitate knowledge capture and distribution in data engineering. He encourages the community to share reference implementations and templates to foster collaboration and innovation, and expresses hopes for a future where code reuse becomes more prevalent. Announcements Hello and welcome to the Data Engineering Podcast, the show about modern data management Data migrations are brutal. They drag on for months—sometimes years—burning through resources and crushing team morale. Datafold's AI-powered Migration Agent changes all that. Their unique combination of AI code translation and automated data validation has helped companies complete migrations up to 10 times faster than manual approaches. And they're so confident in their solution, they'll actually guarantee your timeline in writing. Ready to turn your year-long migration into weeks? Visit dataengineeringpodcast.com/datafold today for the details. Your host is Tobias Macey and today I'm joined again by Max Beauchemin to talk about the challenges of reusability in data pipelines Interview Introduction How did you get involved in the area of data management? Can you start by sharing your current thesis on the opportunities and shortcomings of code and component reusability in the data context? What are some ways that you think about what constitutes a "component" in this context? The data ecosystem has arguably grown more varied and nuanced in recent years. At the same time, the number and maturity of tools has grown. What is your view on the current trend in productivity for data teams and practitioners? What do you see as the core impediments to building more reusable and general-purpose solutions in data engineering? How can we balance the actual needs of data consumers against their requests (whether well- or un-informed) to help increase our ability to better design our workflows for reuse? In data engineering there are two broad approaches; code-focused or SQL-focused pipelines. In principle one would think that code-focused environments would have better composability. What are you seeing as the realities in your personal experience and what you hear from other teams? When it comes to SQL dialects, dbt offers the option of Jinja macros, whereas SDF and SQLMesh offer automatic translation. There are also tools like PRQL and Malloy that aim to abstract away the underlying SQL. What are the tradeoffs across those options that help or hinder the portability of transformation logic? Which layers of the data stack/steps in the data journey do you see the greatest opportunity for improving the creation of more broadly usable abstractions/reusable elements? low/no code systems for code reuse impact of LLMs on reusability/composition impact of background on industry practices (e.g. DBAs, sysadmins, analysts vs. SWE, etc.) polymorphic data models (e.g. activity schema) What are the most interesting, innovative, or unexpected ways that you have seen teams address composability and reusability of data components? What are the most interesting, unexpected, or challenging lessons that you have learned while working on data-oriented tools and utilities? What are your hopes and predictions for sharing of code and logic in the future of data engineering? Contact Info LinkedIn Parting Question From your perspective, what is the biggest gap in the tooling or technology for data management today? Closing Announcements Thank you for listening! Don't forget to check out our other shows. Podcast.__init__ covers the Python language, its community, and the innovative ways it is being used. The AI Engineering Podcast is your guide to the fast-moving world of building AI systems. Visit the site to subscribe to the show, sign up for the mailing list, and read the show notes. If you've learned something or tried out a project from the show then tell us about it! Email hosts@dataengineeringpodcast.com with your story. Links Max's Blog Post Airflow Superset Tableau Looker PowerBI Cohort Analysis NextJS Airbyte Podcast Episode Fivetran Podcast Episode Segment dbt SQLMesh Podcast Episode Spark LAMP Stack PHP Relational Algebra Knowledge Graph Python Marshmallow Data Warehouse Lifecycle Toolkit (affiliate link) Entity Centric Data Modeling Blog Post Amplitude OSACon presentation ol-data-platform Tobias' team's data platform code The intro and outro music is from The Hug by The Freak Fandango Orchestra / CC BY-SA…
D
Data Engineering Podcast

Summary In this episode of the Data Engineering Podcast Sam Kleinman talks about the pivotal role of databases in software engineering. Sam shares his journey into the world of data and discusses the complexities of database selection, highlighting the trade-offs between different database architectures and how these choices affect system design, query performance, and the need for ETL processes. He emphasizes the importance of understanding specific requirements to choose the right database engine and warns against over-engineering solutions that can lead to increased complexity. Sam also touches on the tendency of engineers to move logic to the application layer due to skepticism about database longevity and advises teams to leverage database capabilities instead. Finally, he identifies a significant gap in data management tooling: the lack of easy-to-use testing tools for database interactions, highlighting the need for better testing paradigms to ensure reliability and reduce bugs in data-driven applications. Announcements Hello and welcome to the Data Engineering Podcast, the show about modern data management It’s 2024, why are we still doing data migrations by hand? Teams spend months—sometimes years—manually converting queries and validating data, burning resources and crushing morale. Datafold's AI-powered Migration Agent brings migrations into the modern era. Their unique combination of AI code translation and automated data validation has helped companies complete migrations up to 10 times faster than manual approaches. And they're so confident in their solution, they'll actually guarantee your timeline in writing. Ready to turn your year-long migration into weeks? Visit dataengineeringpodcast.com/datafold today to learn how Datafold can automate your migration and ensure source to target parity. Your host is Tobias Macey and today I'm interviewing Sam Kleinman about database tradeoffs across operating environments and axes of scale Interview Introduction How did you get involved in the area of data management? The database engine you use has a substantial impact on how you architect your overall system. When starting a greenfield project, what do you see as the most important factor to consider when selecting a database? points of friction introduced by database capabilities embedded databases (e.g. SQLite, DuckDB, LanceDB), when to use and when do they become a bottleneck single-node database engines (e.g. Postgres, MySQL), when are they legitimately a problem distributed databases (e.g. CockroachDB, PlanetScale, MongoDB) polyglot storage vs. general-purpose/multimodal databases federated queries, benefits and limitations ease of integration vs. variability of performance and access control Contact Info LinkedIn GitHub Parting Question From your perspective, what is the biggest gap in the tooling or technology for data management today? Closing Announcements Thank you for listening! Don't forget to check out our other shows. Podcast.__init__ covers the Python language, its community, and the innovative ways it is being used. The AI Engineering Podcast is your guide to the fast-moving world of building AI systems. Visit the site to subscribe to the show, sign up for the mailing list, and read the show notes. If you've learned something or tried out a project from the show then tell us about it! Email hosts@dataengineeringpodcast.com with your story. Links MongoDB Neon Podcast Episode GlareDB NoSQL S3 Conditional Write Event driven architecture CockroachDB Couchbase Cassandra The intro and outro music is from The Hug by The Freak Fandango Orchestra / CC BY-SA…
D
Data Engineering Podcast

Summary This episode features an insightful conversation with Petr Janda, the CEO and founder of Synq. Petr shares his journey from being an engineer to founding Synq, emphasizing the importance of treating data systems with the same rigor as engineering systems. He discusses the challenges and solutions in data reliability, including the need for transparency and ownership in data systems. Synq's platform helps data teams manage incidents, understand data dependencies, and ensure data quality by providing insights and automation capabilities. Petr emphasizes the need for a holistic approach to data reliability, integrating data systems into broader business processes. He highlights the role of data teams in modern organizations and how Synq is empowering them to achieve this. Announcements Hello and welcome to the Data Engineering Podcast, the show about modern data management Data lakes are notoriously complex. For data engineers who battle to build and scale high quality data workflows on the data lake, Starburst is an end-to-end data lakehouse platform built on Trino, the query engine Apache Iceberg was designed for, with complete support for all table formats including Apache Iceberg, Hive, and Delta Lake. Trusted by teams of all sizes, including Comcast and Doordash. Want to see Starburst in action? Go to dataengineeringpodcast.com/starburst and get $500 in credits to try Starburst Galaxy today, the easiest and fastest way to get started using Trino. Your host is Tobias Macey and today I'm interviewing Petr Janda about Synq, a data reliability platform focused on leveling up data teams by supporting a culture of engineering rigor Interview Introduction How did you get involved in the area of data management? Can you describe what Synq is and the story behind it? Data observability/reliability is a category that grew rapidly over the past ~5 years and has several vendors focused on different elements of the problem. What are the capabilities that you saw as lacking in the ecosystem which you are looking to address? Operational/infrastructure engineers have spent the past decade honing their approach to incident management and uptime commitments. How do those concepts map to the responsibilities and workflows of data teams? Tooling only plays a small part in SLAs and incident management. How does Synq help to support the cultural transformation that is necessary? What does an on-call rotation for a data engineer/data platform engineer look like as compared with an application-focused team? How does the focus on data assets/data products shift your approach to observability as compared to a table/pipeline centric approach? With the focus on sharing ownership beyond the boundaries on the data team there is a strong correlation with data governance principles. How do you see organizations incorporating Synq into their approach to data governance/compliance? Can you describe how Synq is designed/implemented? How have the scope and goals of the product changed since you first started working on it? For a team who is onboarding onto Synq, what are the steps required to get it integrated into their technology stack and workflows? What are the types of incidents/errors that you are able to identify and alert on? What does a typical incident/error resolution process look like with Synq? What are the most interesting, innovative, or unexpected ways that you have seen Synq used? What are the most interesting, unexpected, or challenging lessons that you have learned while working on Synq? When is Synq the wrong choice? What do you have planned for the future of Synq? Contact Info LinkedIn Substack Parting Question From your perspective, what is the biggest gap in the tooling or technology for data management today? Closing Announcements Thank you for listening! Don't forget to check out our other shows. Podcast.__init__ covers the Python language, its community, and the innovative ways it is being used. The Machine Learning Podcast helps you go from idea to production with machine learning. Visit the site to subscribe to the show, sign up for the mailing list, and read the show notes. If you've learned something or tried out a project from the show then tell us about it! Email hosts@dataengineeringpodcast.com with your story. Links Synq Incident Management SLA == Service Level Agreement Data Governance Podcast Episode PagerDuty OpsGenie Clickhouse Podcast Episode dbt Podcast Episode SQLMesh Podcast Episode The intro and outro music is from The Hug by The Freak Fandango Orchestra / CC BY-SA…
D
Data Engineering Podcast

Summary Data lakehouse architectures have been gaining significant adoption. To accelerate adoption in the enterprise Microsoft has created the Fabric platform, based on their OneLake architecture. In this episode Dipti Borkar shares her experiences working on the product team at Fabric and explains the various use cases for the Fabric service. Announcements Hello and welcome to the Data Engineering Podcast, the show about modern data management Data lakes are notoriously complex. For data engineers who battle to build and scale high quality data workflows on the data lake, Starburst is an end-to-end data lakehouse platform built on Trino, the query engine Apache Iceberg was designed for, with complete support for all table formats including Apache Iceberg, Hive, and Delta Lake. Trusted by teams of all sizes, including Comcast and Doordash. Want to see Starburst in action? Go to dataengineeringpodcast.com/starburst and get $500 in credits to try Starburst Galaxy today, the easiest and fastest way to get started using Trino. Your host is Tobias Macey and today I'm interviewing Dipti Borkar about her work on Microsoft Fabric and performing analytics on data withou Interview Introduction How did you get involved in the area of data management? Can you describe what Microsoft Fabric is and the story behind it? Data lakes in various forms have been gaining significant popularity as a unified interface to an organization's analytics. What are the motivating factors that you see for that trend? Microsoft has been investing heavily in open source in recent years, and the Fabric platform relies on several open components. What are the benefits of layering on top of existing technologies rather than building a fully custom solution? What are the elements of Fabric that were engineered specifically for the service? What are the most interesting/complicated integration challenges? How has your prior experience with Ahana and Presto informed your current work at Microsoft? AI plays a substantial role in the product. What are the benefits of embedding Copilot into the data engine? What are the challenges in terms of safety and reliability? What are the most interesting, innovative, or unexpected ways that you have seen the Fabric platform used? What are the most interesting, unexpected, or challenging lessons that you have learned while working on data lakes generally, and Fabric specifically? When is Fabric the wrong choice? What do you have planned for the future of data lake analytics? Contact Info LinkedIn Parting Question From your perspective, what is the biggest gap in the tooling or technology for data management today? Closing Announcements Thank you for listening! Don't forget to check out our other shows. Podcast.__init__ covers the Python language, its community, and the innovative ways it is being used. The Machine Learning Podcast helps you go from idea to production with machine learning. Visit the site to subscribe to the show, sign up for the mailing list, and read the show notes. If you've learned something or tried out a project from the show then tell us about it! Email hosts@dataengineeringpodcast.com with your story. Links Microsoft Fabric Ahana episode DB2 Distributed Spark Presto Azure Data MAD Landscape Podcast Episode ML Podcast Episode Tableau dbt Medallion Architecture Microsoft Onelake ORC Parquet Avro Delta Lake Iceberg Podcast Episode Hudi Podcast Episode Hadoop PowerBI Podcast Episode Velox Gluten Apache XTable GraphQL Formula 1 McLaren The intro and outro music is from The Hug by The Freak Fandango Orchestra / CC BY-SA Sponsored By: Starburst : 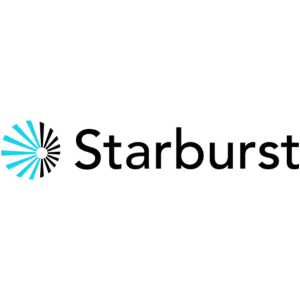 This episode is brought to you by Starburst - an end-to-end data lakehouse platform for data engineers who are battling to build and scale high quality data pipelines on the data lake. Powered by Trino, the query engine Apache Iceberg was designed for, Starburst is an open platform with support for all table formats including Apache Iceberg, Hive, and Delta Lake. Trusted by the teams at Comcast and Doordash, Starburst delivers the adaptability and flexibility a lakehouse ecosystem promises, while providing a single point of access for your data and all your data governance allowing you to discover, transform, govern, and secure all in one place. Want to see Starburst in action? Try Starburst Galaxy today, the easiest and fastest way to get started using Trino, and get $500 of credits free. Go to [dataengineeringpodcast.com/starburst](https://www.dataengineeringpodcast.com/starburst) Support Data Engineering Podcast…
D
Data Engineering Podcast

Summary Stripe is a company that relies on data to power their products and business. To support that functionality they have invested in Trino and Iceberg for their analytical workloads. In this episode Kevin Liu shares some of the interesting features that they have built by combining those technologies, as well as the challenges that they face in supporting the myriad workloads that are thrown at this layer of their data platform. Announcements Hello and welcome to the Data Engineering Podcast, the show about modern data management Data lakes are notoriously complex. For data engineers who battle to build and scale high quality data workflows on the data lake, Starburst is an end-to-end data lakehouse platform built on Trino, the query engine Apache Iceberg was designed for, with complete support for all table formats including Apache Iceberg, Hive, and Delta Lake. Trusted by teams of all sizes, including Comcast and Doordash. Want to see Starburst in action? Go to dataengineeringpodcast.com/starburst and get $500 in credits to try Starburst Galaxy today, the easiest and fastest way to get started using Trino. Your host is Tobias Macey and today I'm interviewing Kevin Liu about his use of Trino and Iceberg for Stripe's data lakehouse Interview Introduction How did you get involved in the area of data management? Can you describe what role Trino and Iceberg play in Stripe's data architecture? What are the ways in which your job responsibilities intersect with Stripe's lakehouse infrastructure? What were the requirements and selection criteria that led to the selection of that combination of technologies? What are the other systems that feed into and rely on the Trino/Iceberg service? what kinds of questions are you answering with table metadata what use case/team does that support comparative utility of iceberg REST catalog What are the shortcomings of Trino and Iceberg? What are the most interesting, innovative, or unexpected ways that you have seen Iceberg/Trino used? What are the most interesting, unexpected, or challenging lessons that you have learned while working on Stripe's data infrastructure? When is a lakehouse on Trino/Iceberg the wrong choice? What do you have planned for the future of Trino and Iceberg at Stripe? Contact Info Substack LinkedIn Parting Question From your perspective, what is the biggest gap in the tooling or technology for data management today? Closing Announcements Thank you for listening! Don't forget to check out our other shows. Podcast.__init__ covers the Python language, its community, and the innovative ways it is being used. The Machine Learning Podcast helps you go from idea to production with machine learning. Visit the site to subscribe to the show, sign up for the mailing list, and read the show notes. If you've learned something or tried out a project from the show then tell us about it! Email hosts@dataengineeringpodcast.com with your story. Links Trino Iceberg Stripe Spark Redshift Hive Metastore Python Iceberg Python Iceberg REST Catalog Trino Metadata Table Flink Podcast Episode Tabular Podcast Episode Delta Table Podcast Episode Databricks Unity Catalog Starburst AWS Athena Kevin Trinofest Presentation Alluxio Podcast Episode Parquet Hudi Trino Project Tardigrade Trino On Ice The intro and outro music is from The Hug by The Freak Fandango Orchestra / CC BY-SA Sponsored By: Starburst : 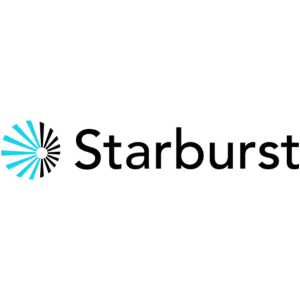 This episode is brought to you by Starburst - an end-to-end data lakehouse platform for data engineers who are battling to build and scale high quality data pipelines on the data lake. Powered by Trino, the query engine Apache Iceberg was designed for, Starburst is an open platform with support for all table formats including Apache Iceberg, Hive, and Delta Lake. Trusted by the teams at Comcast and Doordash, Starburst delivers the adaptability and flexibility a lakehouse ecosystem promises, while providing a single point of access for your data and all your data governance allowing you to discover, transform, govern, and secure all in one place. Want to see Starburst in action? Try Starburst Galaxy today, the easiest and fastest way to get started using Trino, and get $500 of credits free. Go to [dataengineeringpodcast.com/starburst](https://www.dataengineeringpodcast.com/starburst) Support Data Engineering Podcast…
D
Data Engineering Podcast

Summary Streaming data processing enables new categories of data products and analytics. Unfortunately, reasoning about stream processing engines is complex and lacks sufficient tooling. To address this shortcoming Datorios created an observability platform for Flink that brings visibility to the internals of this popular stream processing system. In this episode Ronen Korman and Stav Elkayam discuss how the increased understanding provided by purpose built observability improves the usefulness of Flink. Announcements Hello and welcome to the Data Engineering Podcast, the show about modern data management This episode is supported by Code Comments, an original podcast from Red Hat. As someone who listens to the Data Engineering Podcast, you know that the road from tool selection to production readiness is anything but smooth or straight. In Code Comments, host Jamie Parker, Red Hatter and experienced engineer, shares the journey of technologists from across the industry and their hard-won lessons in implementing new technologies. I listened to the recent episode "Transforming Your Database" and appreciated the valuable advice on how to approach the selection and integration of new databases in applications and the impact on team dynamics. There are 3 seasons of great episodes and new ones landing everywhere you listen to podcasts. Search for "Code Commentst" in your podcast player or go to dataengineeringpodcast.com/codecomments today to subscribe. My thanks to the team at Code Comments for their support. Data lakes are notoriously complex. For data engineers who battle to build and scale high quality data workflows on the data lake, Starburst is an end-to-end data lakehouse platform built on Trino, the query engine Apache Iceberg was designed for, with complete support for all table formats including Apache Iceberg, Hive, and Delta Lake. Trusted by teams of all sizes, including Comcast and Doordash. Want to see Starburst in action? Go to dataengineeringpodcast.com/starburst and get $500 in credits to try Starburst Galaxy today, the easiest and fastest way to get started using Trino. Your host is Tobias Macey and today I'm interviewing Ronen Korman and Stav Elkayam about pulling back the curtain on your real-time data streams by bringing intuitive observability to Flink streams Interview Introduction How did you get involved in the area of data management? Can you describe what Datorios is and the story behind it? Data observability has been gaining adoption for a number of years now, with a large focus on data warehouses. What are some of the unique challenges posed by Flink? How much of the complexity is due to the nature of streaming data vs. the architectural realities of Flink? How has the lack of visibility into the flow of data in Flink impacted the ways that teams think about where/when/how to apply it? How have the requirements of generative AI shifted the demand for streaming data systems? What role does Flink play in the architecture of generative AI systems? Can you describe how Datorios is implemented? How has the design and goals of Datorios changed since you first started working on it? How much of the Datorios architecture and functionality is specific to Flink and how are you thinking about its potential application to other streaming platforms? Can you describe how Datorios is used in a day-to-day workflow for someone building streaming applications on Flink? What are the most interesting, innovative, or unexpected ways that you have seen Datorios used? What are the most interesting, unexpected, or challenging lessons that you have learned while working on Datorios? When is Datorios the wrong choice? What do you have planned for the future of Datorios? Contact Info Ronen LinkedIn Stav LinkedIn Parting Question From your perspective, what is the biggest gap in the tooling or technology for data management today? Closing Announcements Thank you for listening! Don't forget to check out our other shows. Podcast.__init__ covers the Python language, its community, and the innovative ways it is being used. The Machine Learning Podcast helps you go from idea to production with machine learning. Visit the site to subscribe to the show, sign up for the mailing list, and read the show notes. If you've learned something or tried out a project from the show then tell us about it! Email hosts@dataengineeringpodcast.com with your story. Links Datorios Apache Flink Podcast Episode ChatGPT-4o The intro and outro music is from The Hug by The Freak Fandango Orchestra / CC BY-SA Sponsored By: Starburst : 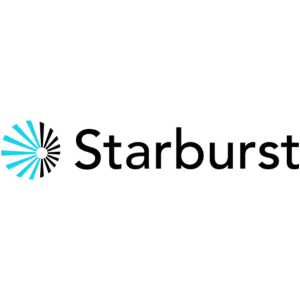 This episode is brought to you by Starburst - an end-to-end data lakehouse platform for data engineers who are battling to build and scale high quality data pipelines on the data lake. Powered by Trino, the query engine Apache Iceberg was designed for, Starburst is an open platform with support for all table formats including Apache Iceberg, Hive, and Delta Lake. Trusted by the teams at Comcast and Doordash, Starburst delivers the adaptability and flexibility a lakehouse ecosystem promises, while providing a single point of access for your data and all your data governance allowing you to discover, transform, govern, and secure all in one place. Want to see Starburst in action? Try Starburst Galaxy today, the easiest and fastest way to get started using Trino, and get $500 of credits free. Go to [dataengineeringpodcast.com/starburst](https://www.dataengineeringpodcast.com/starburst) Red Hat Code Comments Podcast : 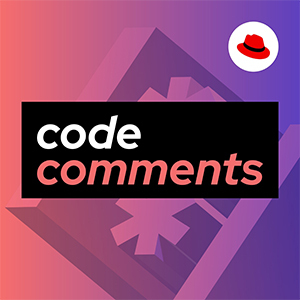 Putting new technology to use is an exciting prospect. But going from purchase to production isn’t always smooth—even when it’s something everyone is looking forward to. Code Comments covers the bumps, the hiccups, and the setbacks teams face when adjusting to new technology—and the triumphs they pull off once they really get going. Follow Code Comments [anywhere you listen to podcasts](https://link.chtbl.com/codecomments?sid=podcast.dataengineering) . Support Data Engineering Podcast…
Summary Modern businesses aspire to be data driven, and technologists enjoy working through the challenge of building data systems to support that goal. Data governance is the binding force between these two parts of the organization. Nicola Askham found her way into data governance by accident, and stayed because of the benefit that she was able to provide by serving as a bridge between the technology and business. In this episode she shares the practical steps to implementing a data governance practice in your organization, and the pitfalls to avoid. Announcements Hello and welcome to the Data Engineering Podcast, the show about modern data management Data lakes are notoriously complex. For data engineers who battle to build and scale high quality data workflows on the data lake, Starburst is an end-to-end data lakehouse platform built on Trino, the query engine Apache Iceberg was designed for, with complete support for all table formats including Apache Iceberg, Hive, and Delta Lake. Trusted by teams of all sizes, including Comcast and Doordash. Want to see Starburst in action? Go to dataengineeringpodcast.com/starburst and get $500 in credits to try Starburst Galaxy today, the easiest and fastest way to get started using Trino. This episode is supported by Code Comments, an original podcast from Red Hat. As someone who listens to the Data Engineering Podcast, you know that the road from tool selection to production readiness is anything but smooth or straight. In Code Comments, host Jamie Parker, Red Hatter and experienced engineer, shares the journey of technologists from across the industry and their hard-won lessons in implementing new technologies. I listened to the recent episode "Transforming Your Database" and appreciated the valuable advice on how to approach the selection and integration of new databases in applications and the impact on team dynamics. There are 3 seasons of great episodes and new ones landing everywhere you listen to podcasts. Search for "Code Commentst" in your podcast player or go to dataengineeringpodcast.com/codecomments today to subscribe. My thanks to the team at Code Comments for their support. Your host is Tobias Macey and today I'm interviewing Nicola Askham about the practical steps of building out a data governance practice in your organization Interview Introduction How did you get involved in the area of data management? Can you start by giving an overview of the scope and boundaries of data governance in an organization? At what point does a lack of an explicit governance policy become a liability? What are some of the misconceptions that you encounter about data governance? What impact has the evolution of data technologies had on the implementation of governance practices? (e.g. number/scale of systems, types of data, AI) Data governance can often become an exercise in boiling the ocean. What are the concrete first steps that will increase the success rate of a governance practice? Once a data governance project is underway, what are some of the common roadblocks that might derail progress? What are the net benefits to the data team and the organization when a data governance practice is established, active, and healthy? What are the most interesting, innovative, or unexpected ways that you have seen data governance applied? What are the most interesting, unexpected, or challenging lessons that you have learned while working on data governance/training/coaching? What are some of the pitfalls in data governance? What are some of the future trends in data governance that you are excited by? Are there any trends that concern you? Contact Info Website LinkedIn Parting Question From your perspective, what is the biggest gap in the tooling or technology for data management today? Closing Announcements Thank you for listening! Don't forget to check out our other shows. Podcast.__init__ covers the Python language, its community, and the innovative ways it is being used. The Machine Learning Podcast helps you go from idea to production with machine learning. Visit the site to subscribe to the show, sign up for the mailing list, and read the show notes. If you've learned something or tried out a project from the show then tell us about it! Email hosts@dataengineeringpodcast.com ) with your story. Links Website Master Data Management Cartesian Join DAMA == Data Management Community DMBOK == Data Management Body of Knowledge DAMA DMBOK Wheel CDMP (Certified Data Management Professional) Exam Data Mesh Data Governance First Steps Checklist The Never Normal The intro and outro music is from The Hug by The Freak Fandango Orchestra / CC BY-SA Sponsored By: Red Hat Code Comments Podcast : 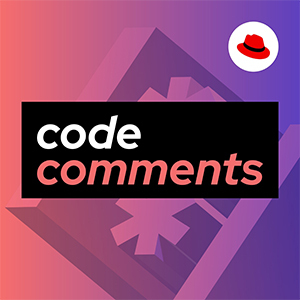 Putting new technology to use is an exciting prospect. But going from purchase to production isn’t always smooth—even when it’s something everyone is looking forward to. Code Comments covers the bumps, the hiccups, and the setbacks teams face when adjusting to new technology—and the triumphs they pull off once they really get going. Follow Code Comments [anywhere you listen to podcasts](https://link.chtbl.com/codecomments?sid=podcast.dataengineering) . Starburst : 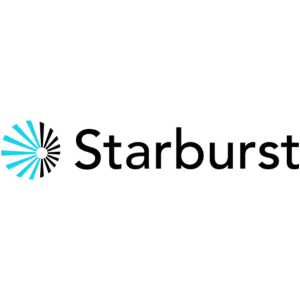 This episode is brought to you by Starburst - an end-to-end data lakehouse platform for data engineers who are battling to build and scale high quality data pipelines on the data lake. Powered by Trino, the query engine Apache Iceberg was designed for, Starburst is an open platform with support for all table formats including Apache Iceberg, Hive, and Delta Lake. Trusted by the teams at Comcast and Doordash, Starburst delivers the adaptability and flexibility a lakehouse ecosystem promises, while providing a single point of access for your data and all your data governance allowing you to discover, transform, govern, and secure all in one place. Want to see Starburst in action? Try Starburst Galaxy today, the easiest and fastest way to get started using Trino, and get $500 of credits free. Go to [dataengineeringpodcast.com/starburst](https://www.dataengineeringpodcast.com/starburst) Support Data Engineering Podcast…
D
Data Engineering Podcast

Summary Any software system that survives long enough will require some form of migration or evolution. When that system is responsible for the data layer the process becomes more challenging. Sriram Panyam has been involved in several projects that required migration of large volumes of data in high traffic environments. In this episode he shares some of the valuable lessons that he learned about how to make those projects successful. Announcements Hello and welcome to the Data Engineering Podcast, the show about modern data management Data lakes are notoriously complex. For data engineers who battle to build and scale high quality data workflows on the data lake, Starburst is an end-to-end data lakehouse platform built on Trino, the query engine Apache Iceberg was designed for, with complete support for all table formats including Apache Iceberg, Hive, and Delta Lake. Trusted by teams of all sizes, including Comcast and Doordash. Want to see Starburst in action? Go to dataengineeringpodcast.com/starburst and get $500 in credits to try Starburst Galaxy today, the easiest and fastest way to get started using Trino. This episode is supported by Code Comments, an original podcast from Red Hat. As someone who listens to the Data Engineering Podcast, you know that the road from tool selection to production readiness is anything but smooth or straight. In Code Comments, host Jamie Parker, Red Hatter and experienced engineer, shares the journey of technologists from across the industry and their hard-won lessons in implementing new technologies. I listened to the recent episode "Transforming Your Database" and appreciated the valuable advice on how to approach the selection and integration of new databases in applications and the impact on team dynamics. There are 3 seasons of great episodes and new ones landing everywhere you listen to podcasts. Search for "Code Commentst" in your podcast player or go to dataengineeringpodcast.com/codecomments today to subscribe. My thanks to the team at Code Comments for their support. Your host is Tobias Macey and today I'm interviewing Sriram Panyam about his experiences conducting large scale data migrations and the useful strategies that he learned in the process Interview Introduction How did you get involved in the area of data management? Can you start by sharing some of your experiences with data migration projects? As you have gone through successive migration projects, how has that influenced the ways that you think about architecting data systems? How would you categorize the different types and motivations of migrations? How does the motivation for a migration influence the ways that you plan for and execute that work? Can you talk us through one or two specific projects that you have taken part in? Part 1: The Triggers Section 1: Technical Limitations triggering Data Migration Scaling bottlenecks: Performance issues with databases, storage, or network infrastructure Legacy compatibility: Difficulties integrating with modern tools and cloud platforms System upgrades: The need to migrate data during major software changes (e.g., SQL Server version upgrade) Section 2: Types of Migrations for Infrastructure Focus Storage migration: Moving data between systems (HDD to SSD, SAN to NAS, etc.) Data center migration: Physical relocation or consolidation of data centers Virtualization migration: Moving from physical servers to virtual machines (or vice versa) Section 3: Technical Decisions Driving Data Migrations End-of-life support: Forced migration when older software or hardware is sunsetted Security and compliance: Adopting new platforms with better security postures Cost Optimization: Potential savings of cloud vs. on-premise data centers Part 2: Challenges (and Anxieties) Section 1: Technical Challenges Data transformation challenges: Schema changes, complex data mappings Network bandwidth and latency: Transferring large datasets efficiently Performance testing and load balancing: Ensuring new systems can handle the workload Live data consistency: Maintaining data integrity while updates occur in the source system Minimizing Lag: Techniques to reduce delays in replicating changes to the new system Change data capture: Identifying and tracking changes to the source system during migration Section 2: Operational Challenges Minimizing downtime: Strategies for service continuity during migration Change management and rollback plans: Dealing with unexpected issues Technical skills and resources: In-house expertise/data teams/external help Section 3: Security & Compliance Challenges Data encryption and protection: Methods for both in-transit and at-rest data Meeting audit requirements: Documenting data lineage & the chain of custody Managing access controls: Adjusting identity and role-based access to the new systems Part 3: Patterns Section 1: Infrastructure Migration Strategies Lift and shift: Migrating as-is vs. modernization and re-architecting during the move Phased vs. big bang approaches: Tradeoffs in risk vs. disruption Tools and automation: Using specialized software to streamline the process Dual writes: Managing updates to both old and new systems for a time Change data capture (CDC) methods: Log-based vs. trigger-based approaches for tracking changes Data validation & reconciliation: Ensuring consistency between source and target Section 2: Maintaining Performance and Reliability Disaster recovery planning: Failover mechanisms for the new environment Monitoring and alerting: Proactively identifying and addressing issues Capacity planning and forecasting growth to scale the new infrastructure Section 3: Data Consistency and Replication Replication tools - strategies and specialized tooling Data synchronization techniques, eg Pros and cons of different methods (incremental vs. full) Testing/Verification Strategies for validating data correctness in a live environment Implication of large scale systems/environments Comparison of interesting strategies: DBLog, Debezium, Databus, Goldengate etc What are the most interesting, innovative, or unexpected approaches to data migrations that you have seen or participated in? What are the most interesting, unexpected, or challenging lessons that you have learned while working on data migrations? When is a migration the wrong choice? What are the characteristics or features of data technologies and the overall ecosystem that can reduce the burden of data migration in the future? Contact Info LinkedIn Parting Question From your perspective, what is the biggest gap in the tooling or technology for data management today? Closing Announcements Thank you for listening! Don't forget to check out our other shows. Podcast.__init__ covers the Python language, its community, and the innovative ways it is being used. The Machine Learning Podcast helps you go from idea to production with machine learning. Visit the site to subscribe to the show, sign up for the mailing list, and read the show notes. If you've learned something or tried out a project from the show then tell us about it! Email hosts@dataengineeringpodcast.com ) with your story. Links DagKnows Google Cloud Dataflow Seinfeld Risk Management ACL == Access Control List LinkedIn Databus - Change Data Capture Espresso Storage HDFS Kafka Postgres Replication Slots Queueing Theory Apache Beam Debezium Airbyte [Fivetran](fivetran.com) Designing Data Intensive Applications by Martin Kleppman (affiliate link) Vector Databases Pinecone Weaviate LAMP Stack Netflix DBLog The intro and outro music is from The Hug by The Freak Fandango Orchestra / CC BY-SA Sponsored By: Red Hat Code Comments Podcast : 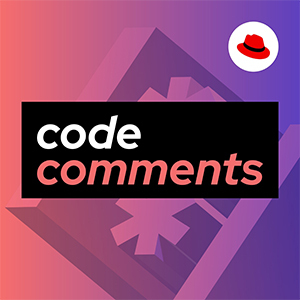 Putting new technology to use is an exciting prospect. But going from purchase to production isn’t always smooth—even when it’s something everyone is looking forward to. Code Comments covers the bumps, the hiccups, and the setbacks teams face when adjusting to new technology—and the triumphs they pull off once they really get going. Follow Code Comments [anywhere you listen to podcasts](https://link.chtbl.com/codecomments?sid=podcast.dataengineering) . Starburst : 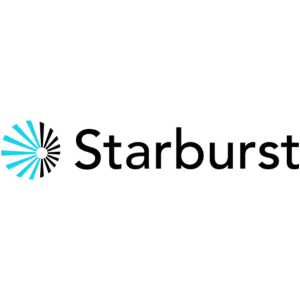 This episode is brought to you by Starburst - an end-to-end data lakehouse platform for data engineers who are battling to build and scale high quality data pipelines on the data lake. Powered by Trino, the query engine Apache Iceberg was designed for, Starburst is an open platform with support for all table formats including Apache Iceberg, Hive, and Delta Lake. Trusted by the teams at Comcast and Doordash, Starburst delivers the adaptability and flexibility a lakehouse ecosystem promises, while providing a single point of access for your data and all your data governance allowing you to discover, transform, govern, and secure all in one place. Want to see Starburst in action? Try Starburst Galaxy today, the easiest and fastest way to get started using Trino, and get $500 of credits free. Go to [dataengineeringpodcast.com/starburst](https://www.dataengineeringpodcast.com/starburst) Support Data Engineering Podcast…
D
Data Engineering Podcast

Summary The purpose of business intelligence systems is to allow anyone in the business to access and decode data to help them make informed decisions. Unfortunately this often turns into an exercise in frustration for everyone involved due to complex workflows and hard-to-understand dashboards. The team at Zenlytic have leaned on the promise of large language models to build an AI agent that lets you converse with your data. In this episode they share their journey through the fast-moving landscape of generative AI and unpack the difference between an AI chatbot and an AI agent. Announcements Hello and welcome to the Data Engineering Podcast, the show about modern data management This episode is supported by Code Comments, an original podcast from Red Hat. As someone who listens to the Data Engineering Podcast, you know that the road from tool selection to production readiness is anything but smooth or straight. In Code Comments, host Jamie Parker, Red Hatter and experienced engineer, shares the journey of technologists from across the industry and their hard-won lessons in implementing new technologies. I listened to the recent episode "Transforming Your Database" and appreciated the valuable advice on how to approach the selection and integration of new databases in applications and the impact on team dynamics. There are 3 seasons of great episodes and new ones landing everywhere you listen to podcasts. Search for "Code Commentst" in your podcast player or go to dataengineeringpodcast.com/codecomments today to subscribe. My thanks to the team at Code Comments for their support. Data lakes are notoriously complex. For data engineers who battle to build and scale high quality data workflows on the data lake, Starburst is an end-to-end data lakehouse platform built on Trino, the query engine Apache Iceberg was designed for, with complete support for all table formats including Apache Iceberg, Hive, and Delta Lake. Trusted by teams of all sizes, including Comcast and Doordash. Want to see Starburst in action? Go to dataengineeringpodcast.com/starburst and get $500 in credits to try Starburst Galaxy today, the easiest and fastest way to get started using Trino. Your host is Tobias Macey and today I'm interviewing Ryan Janssen and Paul Blankley about their experiences building AI powered agents for interacting with your data Interview Introduction How did you get involved in data? In AI? Can you describe what Zenlytic is and the role that AI is playing in your platform? What have been the key stages in your AI journey? What are some of the dead ends that you ran into along the path to where you are today? What are some of the persistent challenges that you are facing? So tell us more about data agents. Firstly, what are data agents and why do you think they're important? How are data agents different from chatbots? Are data agents harder to build? How do you make them work in production? What other technical architectures have you had to develop to support the use of AI in Zenlytic? How have you approached the work of customer education as you introduce this functionality? What are some of the most interesting or erroneous misconceptions that you have heard about what the AI can and can't do? How have you balanced accuracy/trustworthiness with user experience and flexibility in the conversational AI, given the potential for these models to create erroneous responses? What are the most interesting, innovative, or unexpected ways that you have seen your AI agent used? What are the most interesting, unexpected, or challenging lessons that you have learned while working on building an AI agent for business intelligence? When is an AI agent the wrong choice? What do you have planned for the future of AI in the Zenlytic product? Contact Info Ryan LinkedIn Paul LinkedIn Parting Question From your perspective, what is the biggest gap in the tooling or technology for data management today? Closing Announcements Thank you for listening! Don't forget to check out our other shows. Podcast.__init__ covers the Python language, its community, and the innovative ways it is being used. The Machine Learning Podcast helps you go from idea to production with machine learning. Visit the site to subscribe to the show, sign up for the mailing list, and read the show notes. If you've learned something or tried out a project from the show then tell us about it! Email hosts@dataengineeringpodcast.com ) with your story. Links Zenlytic Podcast Episode Attention is all you need Transformers BERT The Bitter Lesson Richard Sutton PID Loops AutoGPT Devin.ai Google Gemini Anthropic Claude OpenAI Code Interpreter Edward Tufte Looker ActionHub OAuth GitHub Copilot The intro and outro music is from The Hug by The Freak Fandango Orchestra / CC BY-SA Sponsored By: Starburst : 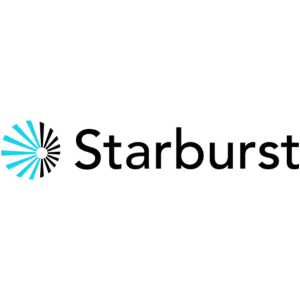 This episode is brought to you by Starburst - an end-to-end data lakehouse platform for data engineers who are battling to build and scale high quality data pipelines on the data lake. Powered by Trino, the query engine Apache Iceberg was designed for, Starburst is an open platform with support for all table formats including Apache Iceberg, Hive, and Delta Lake. Trusted by the teams at Comcast and Doordash, Starburst delivers the adaptability and flexibility a lakehouse ecosystem promises, while providing a single point of access for your data and all your data governance allowing you to discover, transform, govern, and secure all in one place. Want to see Starburst in action? Try Starburst Galaxy today, the easiest and fastest way to get started using Trino, and get $500 of credits free. Go to [dataengineeringpodcast.com/starburst](https://www.dataengineeringpodcast.com/starburst) Red Hat Code Comments Podcast : 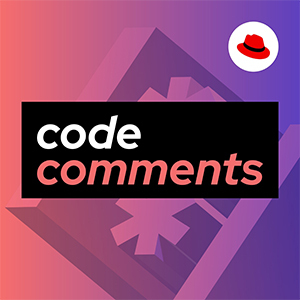 Putting new technology to use is an exciting prospect. But going from purchase to production isn’t always smooth—even when it’s something everyone is looking forward to. Code Comments covers the bumps, the hiccups, and the setbacks teams face when adjusting to new technology—and the triumphs they pull off once they really get going. Follow Code Comments [anywhere you listen to podcasts](https://link.chtbl.com/codecomments?sid=podcast.dataengineering) . Support Data Engineering Podcast…
D
Data Engineering Podcast

Summary Building a data platform is a substrantial engineering endeavor. Once it is running, the next challenge is figuring out how to address release management for all of the different component parts. The services and systems need to be kept up to date, but so does the code that controls their behavior. In this episode your host Tobias Macey reflects on his current challenges in this area and some of the factors that contribute to the complexity of the problem. Announcements Hello and welcome to the Data Engineering Podcast, the show about modern data management This episode is supported by Code Comments, an original podcast from Red Hat. As someone who listens to the Data Engineering Podcast, you know that the road from tool selection to production readiness is anything but smooth or straight. In Code Comments, host Jamie Parker, Red Hatter and experienced engineer, shares the journey of technologists from across the industry and their hard-won lessons in implementing new technologies. I listened to the recent episode "Transforming Your Database" and appreciated the valuable advice on how to approach the selection and integration of new databases in applications and the impact on team dynamics. There are 3 seasons of great episodes and new ones landing everywhere you listen to podcasts. Search for "Code Commentst" in your podcast player or go to dataengineeringpodcast.com/codecomments today to subscribe. My thanks to the team at Code Comments for their support. Data lakes are notoriously complex. For data engineers who battle to build and scale high quality data workflows on the data lake, Starburst is an end-to-end data lakehouse platform built on Trino, the query engine Apache Iceberg was designed for, with complete support for all table formats including Apache Iceberg, Hive, and Delta Lake. Trusted by teams of all sizes, including Comcast and Doordash. Want to see Starburst in action? Go to dataengineeringpodcast.com/starburst and get $500 in credits to try Starburst Galaxy today, the easiest and fastest way to get started using Trino. Your host is Tobias Macey and today I want to talk about my experiences managing the QA and release management process of my data platform Interview Introduction As a team, our overall goal is to ensure that the production environment for our data platform is highly stable and reliable. This is the foundational element of establishing and maintaining trust with the consumers of our data. In order to support this effort, we need to ensure that only changes that have been tested and verified are promoted to production. Our current challenge is one that plagues all data teams. We want to have an environment that mirrors our production environment that is available for testing, but it’s not feasible to maintain a complete duplicate of all of the production data. Compounding that challenge is the fact that each of the components of our data platform interact with data in slightly different ways and need different processes for ensuring that changes are being promoted safely. Contact Info LinkedIn Website Closing Announcements Thank you for listening! Don't forget to check out our other shows. Podcast.__init__ covers the Python language, its community, and the innovative ways it is being used. The Machine Learning Podcast helps you go from idea to production with machine learning. Visit the site to subscribe to the show, sign up for the mailing list, and read the show notes. If you've learned something or tried out a project from the show then tell us about it! Email hosts@dataengineeringpodcast.com with your story. Links Data Platforms and Leaky Abstractions Episode Building A Data Platform From Scratch Airbyte Podcast Episode Trino dbt Starburst Galaxy Superset Dagster LakeFS Podcast Episode Nessie Podcast Episode Iceberg Snowflake LocalStack DSL == Domain Specific Language The intro and outro music is from The Hug by The Freak Fandango Orchestra / CC BY-SA Sponsored By: Starburst : 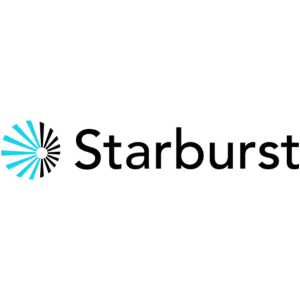 This episode is brought to you by Starburst - an end-to-end data lakehouse platform for data engineers who are battling to build and scale high quality data pipelines on the data lake. Powered by Trino, the query engine Apache Iceberg was designed for, Starburst is an open platform with support for all table formats including Apache Iceberg, Hive, and Delta Lake. Trusted by the teams at Comcast and Doordash, Starburst delivers the adaptability and flexibility a lakehouse ecosystem promises, while providing a single point of access for your data and all your data governance allowing you to discover, transform, govern, and secure all in one place. Want to see Starburst in action? Try Starburst Galaxy today, the easiest and fastest way to get started using Trino, and get $500 of credits free. Go to [dataengineeringpodcast.com/starburst](https://www.dataengineeringpodcast.com/starburst) Red Hat Code Comments Podcast : 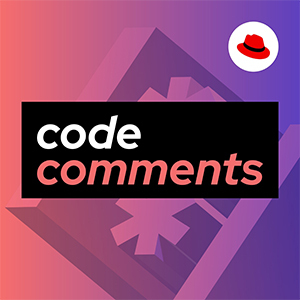 Putting new technology to use is an exciting prospect. But going from purchase to production isn’t always smooth—even when it’s something everyone is looking forward to. Code Comments covers the bumps, the hiccups, and the setbacks teams face when adjusting to new technology—and the triumphs they pull off once they really get going. Follow Code Comments [anywhere you listen to podcasts](https://link.chtbl.com/codecomments?sid=podcast.dataengineering) . Support Data Engineering Podcast…
D
Data Engineering Podcast

Summary Artificial intelligence has dominated the headlines for several months due to the successes of large language models. This has prompted numerous debates about the possibility of, and timeline for, artificial general intelligence (AGI). Peter Voss has dedicated decades of his life to the pursuit of truly intelligent software through the approach of cognitive AI. In this episode he explains his approach to building AI in a more human-like fashion and the emphasis on learning rather than statistical prediction. Announcements Hello and welcome to the Data Engineering Podcast, the show about modern data management Dagster offers a new approach to building and running data platforms and data pipelines. It is an open-source, cloud-native orchestrator for the whole development lifecycle, with integrated lineage and observability, a declarative programming model, and best-in-class testability. Your team can get up and running in minutes thanks to Dagster Cloud, an enterprise-class hosted solution that offers serverless and hybrid deployments, enhanced security, and on-demand ephemeral test deployments. Go to dataengineeringpodcast.com/dagster today to get started. Your first 30 days are free! Data lakes are notoriously complex. For data engineers who battle to build and scale high quality data workflows on the data lake, Starburst powers petabyte-scale SQL analytics fast, at a fraction of the cost of traditional methods, so that you can meet all your data needs ranging from AI to data applications to complete analytics. Trusted by teams of all sizes, including Comcast and Doordash, Starburst is a data lake analytics platform that delivers the adaptability and flexibility a lakehouse ecosystem promises. And Starburst does all of this on an open architecture with first-class support for Apache Iceberg, Delta Lake and Hudi, so you always maintain ownership of your data. Want to see Starburst in action? Go to dataengineeringpodcast.com/starburst and get $500 in credits to try Starburst Galaxy today, the easiest and fastest way to get started using Trino. Your host is Tobias Macey and today I'm interviewing Peter Voss about what is involved in making your AI applications more "human" Interview Introduction How did you get involved in machine learning? Can you start by unpacking the idea of "human-like" AI? How does that contrast with the conception of "AGI"? The applications and limitations of GPT/LLM models have been dominating the popular conversation around AI. How do you see that impacting the overrall ecosystem of ML/AI applications and investment? The fundamental/foundational challenge of every AI use case is sourcing appropriate data. What are the strategies that you have found useful to acquire, evaluate, and prepare data at an appropriate scale to build high quality models? What are the opportunities and limitations of causal modeling techniques for generalized AI models? As AI systems gain more sophistication there is a challenge with establishing and maintaining trust. What are the risks involved in deploying more human-level AI systems and monitoring their reliability? What are the practical/architectural methods necessary to build more cognitive AI systems? How would you characterize the ecosystem of tools/frameworks available for creating, evolving, and maintaining these applications? What are the most interesting, innovative, or unexpected ways that you have seen cognitive AI applied? What are the most interesting, unexpected, or challenging lessons that you have learned while working on desiging/developing cognitive AI systems? When is cognitive AI the wrong choice? What do you have planned for the future of cognitive AI applications at Aigo? Contact Info LinkedIn Website Parting Question From your perspective, what is the biggest barrier to adoption of machine learning today? Closing Announcements Thank you for listening! Don't forget to check out our other shows. Podcast.__init__ covers the Python language, its community, and the innovative ways it is being used. The Machine Learning Podcast helps you go from idea to production with machine learning. Visit the site to subscribe to the show, sign up for the mailing list, and read the show notes. If you've learned something or tried out a project from the show then tell us about it! Email hosts@dataengineeringpodcast.com ) with your story. Links Aigo.ai Artificial General Intelligence Cognitive AI Knowledge Graph Causal Modeling Bayesian Statistics Thinking Fast & Slow by Daniel Kahneman (affiliate link) Agent-Based Modeling Reinforcement Learning DARPA 3 Waves of AI presentation Why Don't We Have AGI Yet? whitepaper Concepts Is All You Need Whitepaper Hellen Keller Stephen Hawking The intro and outro music is from Hitman's Lovesong feat. Paola Graziano by The Freak Fandango Orchestra / CC BY-SA 3.0…
D
Data Engineering Podcast

Summary Generative AI promises to accelerate the productivity of human collaborators. Currently the primary way of working with these tools is through a conversational prompt, which is often cumbersome and unwieldy. In order to simplify the integration of AI capabilities into developer workflows Tsavo Knott helped create Pieces, a powerful collection of tools that complements the tools that developers already use. In this episode he explains the data collection and preparation process, the collection of model types and sizes that work together to power the experience, and how to incorporate it into your workflow to act as a second brain. Announcements Hello and welcome to the Data Engineering Podcast, the show about modern data management Dagster offers a new approach to building and running data platforms and data pipelines. It is an open-source, cloud-native orchestrator for the whole development lifecycle, with integrated lineage and observability, a declarative programming model, and best-in-class testability. Your team can get up and running in minutes thanks to Dagster Cloud, an enterprise-class hosted solution that offers serverless and hybrid deployments, enhanced security, and on-demand ephemeral test deployments. Go to dataengineeringpodcast.com/dagster today to get started. Your first 30 days are free! Data lakes are notoriously complex. For data engineers who battle to build and scale high quality data workflows on the data lake, Starburst powers petabyte-scale SQL analytics fast, at a fraction of the cost of traditional methods, so that you can meet all your data needs ranging from AI to data applications to complete analytics. Trusted by teams of all sizes, including Comcast and Doordash, Starburst is a data lake analytics platform that delivers the adaptability and flexibility a lakehouse ecosystem promises. And Starburst does all of this on an open architecture with first-class support for Apache Iceberg, Delta Lake and Hudi, so you always maintain ownership of your data. Want to see Starburst in action? Go to dataengineeringpodcast.com/starburst and get $500 in credits to try Starburst Galaxy today, the easiest and fastest way to get started using Trino. Your host is Tobias Macey and today I'm interviewing Tsavo Knott about Pieces, a personal AI toolkit to improve the efficiency of developers Interview Introduction How did you get involved in machine learning? Can you describe what Pieces is and the story behind it? The past few months have seen an endless series of personalized AI tools launched. What are the features and focus of Pieces that might encourage someone to use it over the alternatives? model selections architecture of Pieces application local vs. hybrid vs. online models model update/delivery process data preparation/serving for models in context of Pieces app application of AI to developer workflows types of workflows that people are building with pieces What are the most interesting, innovative, or unexpected ways that you have seen Pieces used? What are the most interesting, unexpected, or challenging lessons that you have learned while working on Pieces? When is Pieces the wrong choice? What do you have planned for the future of Pieces? Contact Info LinkedIn Parting Question From your perspective, what is the biggest barrier to adoption of machine learning today? Closing Announcements Thank you for listening! Don't forget to check out our other shows. Podcast.__init__ covers the Python language, its community, and the innovative ways it is being used. The Machine Learning Podcast helps you go from idea to production with machine learning. Visit the site to subscribe to the show, sign up for the mailing list, and read the show notes. If you've learned something or tried out a project from the show then tell us about it! Email hosts@dataengineeringpodcast.com ) with your story. Links Pieces NPU == Neural Processing Unit Tensor Chip LoRA == Low Rank Adaptation Generative Adversarial Networks Mistral Emacs Vim NeoVim Dart Flutter Typescript Lua Retrieval Augmented Generation ONNX LSTM == Long Short-Term Memory LLama 2 GitHub Copilot Tabnine Podcast Episode The intro and outro music is from Hitman's Lovesong feat. Paola Graziano by The Freak Fandango Orchestra / CC BY-SA 3.0…
D
Data Engineering Podcast

Summary Generative AI has rapidly transformed everything in the technology sector. When Andrew Lee started work on Shortwave he was focused on making email more productive. When AI started gaining adoption he realized that he had even more potential for a transformative experience. In this episode he shares the technical challenges that he and his team have overcome in integrating AI into their product, as well as the benefits and features that it provides to their customers. Announcements Hello and welcome to the Data Engineering Podcast, the show about modern data management Dagster offers a new approach to building and running data platforms and data pipelines. It is an open-source, cloud-native orchestrator for the whole development lifecycle, with integrated lineage and observability, a declarative programming model, and best-in-class testability. Your team can get up and running in minutes thanks to Dagster Cloud, an enterprise-class hosted solution that offers serverless and hybrid deployments, enhanced security, and on-demand ephemeral test deployments. Go to dataengineeringpodcast.com/dagster today to get started. Your first 30 days are free! Data lakes are notoriously complex. For data engineers who battle to build and scale high quality data workflows on the data lake, Starburst powers petabyte-scale SQL analytics fast, at a fraction of the cost of traditional methods, so that you can meet all your data needs ranging from AI to data applications to complete analytics. Trusted by teams of all sizes, including Comcast and Doordash, Starburst is a data lake analytics platform that delivers the adaptability and flexibility a lakehouse ecosystem promises. And Starburst does all of this on an open architecture with first-class support for Apache Iceberg, Delta Lake and Hudi, so you always maintain ownership of your data. Want to see Starburst in action? Go to dataengineeringpodcast.com/starburst and get $500 in credits to try Starburst Galaxy today, the easiest and fastest way to get started using Trino. Your host is Tobias Macey and today I'm interviewing Andrew Lee about his work on Shortwave, an AI powered email client Interview Introduction How did you get involved in the area of data management? Can you describe what Shortwave is and the story behind it? What is the core problem that you are addressing with Shortwave? Email has been a central part of communication and business productivity for decades now. What are the overall themes that continue to be problematic? What are the strengths that email maintains as a protocol and ecosystem? From a product perspective, what are the data challenges that are posed by email? Can you describe how you have architected the Shortwave platform? How have the design and goals of the product changed since you started it? What are the ways that the advent and evolution of language models have influenced your product roadmap? How do you manage the personalization of the AI functionality in your system for each user/team? For users and teams who are using Shortwave, how does it change their workflow and communication patterns? Can you describe how I would use Shortwave for managing the workflow of evaluating, planning, and promoting my podcast episodes? What are the most interesting, innovative, or unexpected ways that you have seen Shortwave used? What are the most interesting, unexpected, or challenging lessons that you have learned while working on Shortwave? When is Shortwave the wrong choice? What do you have planned for the future of Shortwave? Contact Info LinkedIn Blog Parting Question From your perspective, what is the biggest gap in the tooling or technology for data management today? Closing Announcements Thank you for listening! Don't forget to check out our other shows. Podcast.__init__ covers the Python language, its community, and the innovative ways it is being used. The Machine Learning Podcast helps you go from idea to production with machine learning. Visit the site to subscribe to the show, sign up for the mailing list, and read the show notes. If you've learned something or tried out a project from the show then tell us about it! Email hosts@dataengineeringpodcast.com ) with your story. Links Shortwave Firebase Google Inbox Hey Ezra Klein Hey Article Superhuman Pinecone Podcast Episode Elastic Hybrid Search Semantic Search Mistral GPT 3.5 IMAP The intro and outro music is from The Hug by The Freak Fandango Orchestra / CC BY-SA Sponsored By: Starburst : 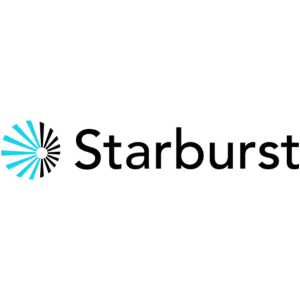 This episode is brought to you by Starburst - a data lake analytics platform for data engineers who are battling to build and scale high quality data pipelines on the data lake. Powered by Trino, Starburst runs petabyte-scale SQL analytics fast at a fraction of the cost of traditional methods, helping you meet all your data needs ranging from AI/ML workloads to data applications to complete analytics. Trusted by the teams at Comcast and Doordash, Starburst delivers the adaptability and flexibility a lakehouse ecosystem promises, while providing a single point of access for your data and all your data governance allowing you to discover, transform, govern, and secure all in one place. Starburst does all of this on an open architecture with first-class support for Apache Iceberg, Delta Lake and Hudi, so you always maintain ownership of your data. Want to see Starburst in action? Try Starburst Galaxy today, the easiest and fastest way to get started using Trino, and get $500 of credits free. [dataengineeringpodcast.com/starburst](https://www.dataengineeringpodcast.com/starburst) Dagster : 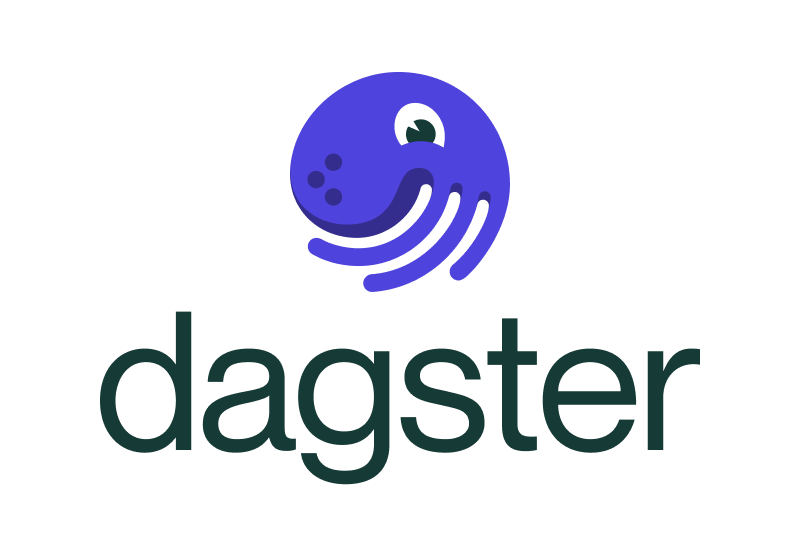 Data teams are tasked with helping organizations deliver on the premise of data, and with ML and AI maturing rapidly, expectations have never been this high. However data engineers are challenged by both technical complexity and organizational complexity, with heterogeneous technologies to adopt, multiple data disciplines converging, legacy systems to support, and costs to manage. Dagster is an open-source orchestration solution that helps data teams reign in this complexity and build data platforms that provide unparalleled observability, and testability, all while fostering collaboration across the enterprise. With enterprise-grade hosting on Dagster Cloud, you gain even more capabilities, adding cost management, security, and CI support to further boost your teams' productivity. Go to [dagster.io](https://dagster.io/lp/dagster-cloud-trial?source=data-eng-podcast) today to get your first 30 days free! Support Data Engineering Podcast…
D
Data Engineering Podcast

Summary Databases come in a variety of formats for different use cases. The default association with the term "database" is relational engines, but non-relational engines are also used quite widely. In this episode Oren Eini, CEO and creator of RavenDB, explores the nuances of relational vs. non-relational engines, and the strategies for designing a non-relational database. Announcements Hello and welcome to the Data Engineering Podcast, the show about modern data management This episode is brought to you by Datafold – a testing automation platform for data engineers that prevents data quality issues from entering every part of your data workflow, from migration to dbt deployment. Datafold has recently launched data replication testing, providing ongoing validation for source-to-target replication. Leverage Datafold's fast cross-database data diffing and Monitoring to test your replication pipelines automatically and continuously. Validate consistency between source and target at any scale, and receive alerts about any discrepancies. Learn more about Datafold by visiting dataengineeringpodcast.com/datafold . Dagster offers a new approach to building and running data platforms and data pipelines. It is an open-source, cloud-native orchestrator for the whole development lifecycle, with integrated lineage and observability, a declarative programming model, and best-in-class testability. Your team can get up and running in minutes thanks to Dagster Cloud, an enterprise-class hosted solution that offers serverless and hybrid deployments, enhanced security, and on-demand ephemeral test deployments. Go to dataengineeringpodcast.com/dagster today to get started. Your first 30 days are free! Data lakes are notoriously complex. For data engineers who battle to build and scale high quality data workflows on the data lake, Starburst powers petabyte-scale SQL analytics fast, at a fraction of the cost of traditional methods, so that you can meet all your data needs ranging from AI to data applications to complete analytics. Trusted by teams of all sizes, including Comcast and Doordash, Starburst is a data lake analytics platform that delivers the adaptability and flexibility a lakehouse ecosystem promises. And Starburst does all of this on an open architecture with first-class support for Apache Iceberg, Delta Lake and Hudi, so you always maintain ownership of your data. Want to see Starburst in action? Go to dataengineeringpodcast.com/starburst and get $500 in credits to try Starburst Galaxy today, the easiest and fastest way to get started using Trino. Your host is Tobias Macey and today I'm interviewing Oren Eini about the work of designing and building a NoSQL database engine Interview Introduction How did you get involved in the area of data management? Can you describe what constitutes a NoSQL database? How have the requirements and applications of NoSQL engines changed since they first became popular ~15 years ago? What are the factors that convince teams to use a NoSQL vs. SQL database? NoSQL is a generalized term that encompasses a number of different data models. How does the underlying representation (e.g. document, K/V, graph) change that calculus? How have the evolution in data formats (e.g. N-dimensional vectors, point clouds, etc.) changed the landscape for NoSQL engines? When designing and building a database, what are the initial set of questions that need to be answered? How many "core capabilities" can you reasonably design around before they conflict with each other? How have you approached the evolution of RavenDB as you add new capabilities and mature the project? What are some of the early decisions that had to be unwound to enable new capabilities? If you were to start from scratch today, what database would you build? What are the most interesting, innovative, or unexpected ways that you have seen RavenDB/NoSQL databases used? What are the most interesting, unexpected, or challenging lessons that you have learned while working on RavenDB? When is a NoSQL database/RavenDB the wrong choice? What do you have planned for the future of RavenDB? Contact Info Blog LinkedIn Parting Question From your perspective, what is the biggest gap in the tooling or technology for data management today? Closing Announcements Thank you for listening! Don't forget to check out our other shows. Podcast.__init__ covers the Python language, its community, and the innovative ways it is being used. The Machine Learning Podcast helps you go from idea to production with machine learning. Visit the site to subscribe to the show, sign up for the mailing list, and read the show notes. If you've learned something or tried out a project from the show then tell us about it! Email hosts@dataengineeringpodcast.com ) with your story. Links RavenDB RSS Object Relational Mapper (ORM) Relational Database NoSQL CouchDB Navigational Database MongoDB Redis Neo4J Cassandra Column-Family SQLite LevelDB Firebird DB fsync Esent DB? KNN == K-Nearest Neighbors RocksDB C# Language ASP.NET QUIC Dynamo Paper Database Internals book (affiliate link) Designing Data Intensive Applications book (affiliate link) The intro and outro music is from The Hug by The Freak Fandango Orchestra / CC BY-SA Sponsored By: Starburst : 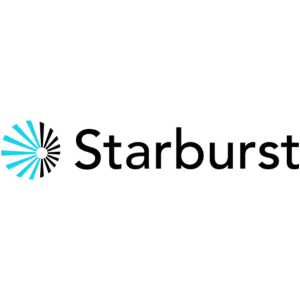 This episode is brought to you by Starburst - a data lake analytics platform for data engineers who are battling to build and scale high quality data pipelines on the data lake. Powered by Trino, Starburst runs petabyte-scale SQL analytics fast at a fraction of the cost of traditional methods, helping you meet all your data needs ranging from AI/ML workloads to data applications to complete analytics. Trusted by the teams at Comcast and Doordash, Starburst delivers the adaptability and flexibility a lakehouse ecosystem promises, while providing a single point of access for your data and all your data governance allowing you to discover, transform, govern, and secure all in one place. Starburst does all of this on an open architecture with first-class support for Apache Iceberg, Delta Lake and Hudi, so you always maintain ownership of your data. Want to see Starburst in action? Try Starburst Galaxy today, the easiest and fastest way to get started using Trino, and get $500 of credits free. [dataengineeringpodcast.com/starburst](https://www.dataengineeringpodcast.com/starburst) Datafold : 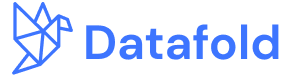 This episode is brought to you by Datafold – a testing automation platform for data engineers that prevents data quality issues from entering every part of your data workflow, from migration to dbt deployment. Datafold has recently launched data replication testing, providing ongoing validation for source-to-target replication. Leverage Datafold's fast cross-database data diffing and Monitoring to test your replication pipelines automatically and continuously. Validate consistency between source and target at any scale, and receive alerts about any discrepancies. Learn more about Datafold by visiting https://get.datafold.com/replication-de-podcast. Dagster : 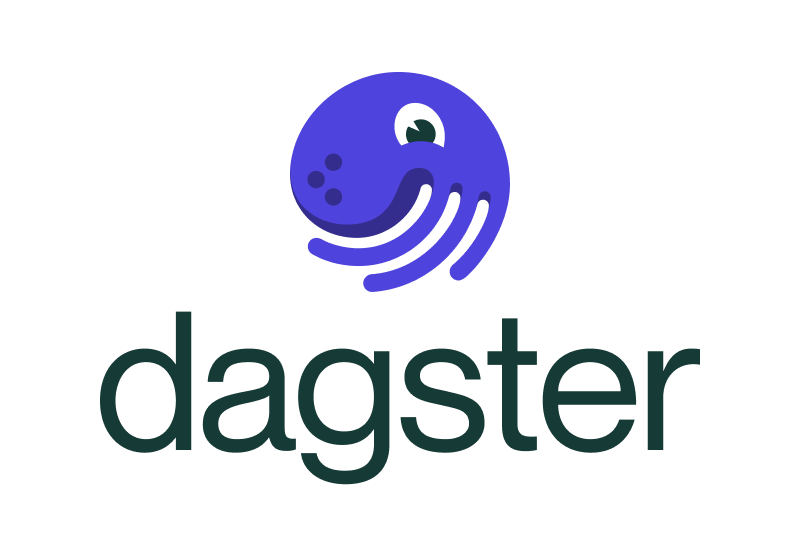 Data teams are tasked with helping organizations deliver on the premise of data, and with ML and AI maturing rapidly, expectations have never been this high. However data engineers are challenged by both technical complexity and organizational complexity, with heterogeneous technologies to adopt, multiple data disciplines converging, legacy systems to support, and costs to manage. Dagster is an open-source orchestration solution that helps data teams reign in this complexity and build data platforms that provide unparalleled observability, and testability, all while fostering collaboration across the enterprise. With enterprise-grade hosting on Dagster Cloud, you gain even more capabilities, adding cost management, security, and CI support to further boost your teams' productivity. Go to [dagster.io](https://dagster.io/lp/dagster-cloud-trial?source=data-eng-podcast) today to get your first 30 days free! Support Data Engineering Podcast…
D
Data Engineering Podcast

Summary Maintaining a single source of truth for your data is the biggest challenge in data engineering. Different roles and tasks in the business need their own ways to access and analyze the data in the organization. In order to enable this use case, while maintaining a single point of access, the semantic layer has evolved as a technological solution to the problem. In this episode Artyom Keydunov, creator of Cube, discusses the evolution and applications of the semantic layer as a component of your data platform, and how Cube provides speed and cost optimization for your data consumers. Announcements Hello and welcome to the Data Engineering Podcast, the show about modern data management This episode is brought to you by Datafold – a testing automation platform for data engineers that prevents data quality issues from entering every part of your data workflow, from migration to dbt deployment. Datafold has recently launched data replication testing, providing ongoing validation for source-to-target replication. Leverage Datafold's fast cross-database data diffing and Monitoring to test your replication pipelines automatically and continuously. Validate consistency between source and target at any scale, and receive alerts about any discrepancies. Learn more about Datafold by visiting dataengineeringpodcast.com/datafold . Dagster offers a new approach to building and running data platforms and data pipelines. It is an open-source, cloud-native orchestrator for the whole development lifecycle, with integrated lineage and observability, a declarative programming model, and best-in-class testability. Your team can get up and running in minutes thanks to Dagster Cloud, an enterprise-class hosted solution that offers serverless and hybrid deployments, enhanced security, and on-demand ephemeral test deployments. Go to dataengineeringpodcast.com/dagster today to get started. Your first 30 days are free! Data lakes are notoriously complex. For data engineers who battle to build and scale high quality data workflows on the data lake, Starburst powers petabyte-scale SQL analytics fast, at a fraction of the cost of traditional methods, so that you can meet all your data needs ranging from AI to data applications to complete analytics. Trusted by teams of all sizes, including Comcast and Doordash, Starburst is a data lake analytics platform that delivers the adaptability and flexibility a lakehouse ecosystem promises. And Starburst does all of this on an open architecture with first-class support for Apache Iceberg, Delta Lake and Hudi, so you always maintain ownership of your data. Want to see Starburst in action? Go to dataengineeringpodcast.com/starburst and get $500 in credits to try Starburst Galaxy today, the easiest and fastest way to get started using Trino. Your host is Tobias Macey and today I'm interviewing Artyom Keydunov about the role of the semantic layer in your data platform Interview Introduction How did you get involved in the area of data management? Can you start by outlining the technical elements of what it means to have a "semantic layer"? In the past couple of years there was a rapid hype cycle around the "metrics layer" and "headless BI", which has largely faded. Can you give your assessment of the current state of the industry around the adoption/implementation of these concepts? What are the benefits of having a discrete service that offers the business metrics/semantic mappings as opposed to implementing those concepts as part of a more general system? (e.g. dbt, BI, warehouse marts, etc.) At what point does it become necessary/beneficial for a team to adopt such a service? What are the challenges involved in retrofitting a semantic layer into a production data system? evolution of requirements/usage patterns technical complexities/performance and cost optimization What are the most interesting, innovative, or unexpected ways that you have seen Cube used? What are the most interesting, unexpected, or challenging lessons that you have learned while working on Cube? When is Cube/a semantic layer the wrong choice? What do you have planned for the future of Cube? Contact Info LinkedIn keydunov on GitHub Parting Question From your perspective, what is the biggest gap in the tooling or technology for data management today? Closing Announcements Thank you for listening! Don't forget to check out our other shows. Podcast.__init__ covers the Python language, its community, and the innovative ways it is being used. The Machine Learning Podcast helps you go from idea to production with machine learning. Visit the site to subscribe to the show, sign up for the mailing list, and read the show notes. If you've learned something or tried out a project from the show then tell us about it! Email hosts@dataengineeringpodcast.com ) with your story. Links Cube Semantic Layer Business Objects Tableau Looker Podcast Episode Mode Thoughtspot LightDash Podcast Episode Embedded Analytics Dimensional Modeling Clickhouse Podcast Episode Druid BigQuery Starburst Pinot Snowflake Podcast Episode Arrow Datafusion Metabase Podcast Episode Superset Alation Collibra Podcast Episode Atlan Podcast Episode The intro and outro music is from The Hug by The Freak Fandango Orchestra / CC BY-SA Sponsored By: Starburst : 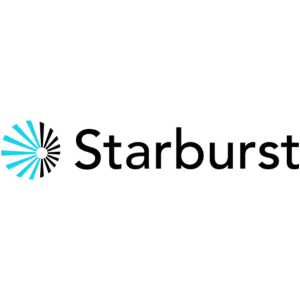 This episode is brought to you by Starburst - a data lake analytics platform for data engineers who are battling to build and scale high quality data pipelines on the data lake. Powered by Trino, Starburst runs petabyte-scale SQL analytics fast at a fraction of the cost of traditional methods, helping you meet all your data needs ranging from AI/ML workloads to data applications to complete analytics. Trusted by the teams at Comcast and Doordash, Starburst delivers the adaptability and flexibility a lakehouse ecosystem promises, while providing a single point of access for your data and all your data governance allowing you to discover, transform, govern, and secure all in one place. Starburst does all of this on an open architecture with first-class support for Apache Iceberg, Delta Lake and Hudi, so you always maintain ownership of your data. Want to see Starburst in action? Try Starburst Galaxy today, the easiest and fastest way to get started using Trino, and get $500 of credits free. [dataengineeringpodcast.com/starburst](https://www.dataengineeringpodcast.com/starburst) Datafold : 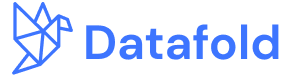 This episode is brought to you by Datafold – a testing automation platform for data engineers that prevents data quality issues from entering every part of your data workflow, from migration to dbt deployment. Datafold has recently launched data replication testing, providing ongoing validation for source-to-target replication. Leverage Datafold's fast cross-database data diffing and Monitoring to test your replication pipelines automatically and continuously. Validate consistency between source and target at any scale, and receive alerts about any discrepancies. Learn more about Datafold by visiting https://get.datafold.com/replication-de-podcast. Dagster : 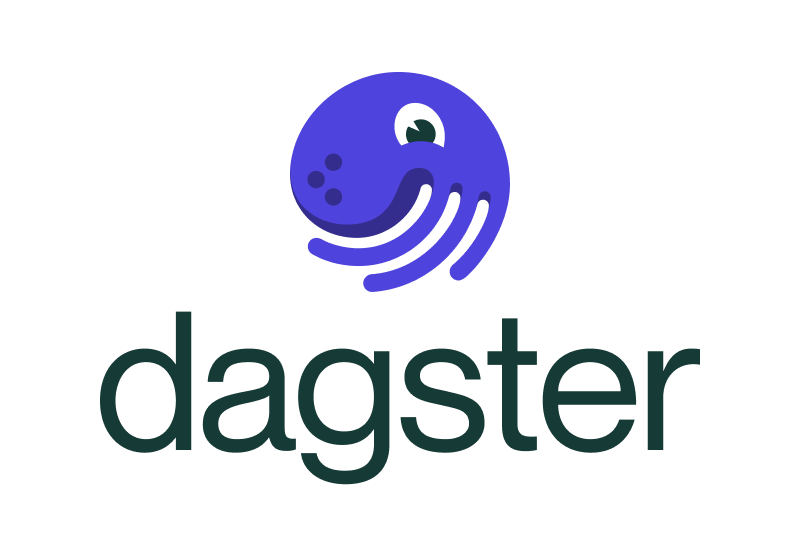 Data teams are tasked with helping organizations deliver on the premise of data, and with ML and AI maturing rapidly, expectations have never been this high. However data engineers are challenged by both technical complexity and organizational complexity, with heterogeneous technologies to adopt, multiple data disciplines converging, legacy systems to support, and costs to manage. Dagster is an open-source orchestration solution that helps data teams reign in this complexity and build data platforms that provide unparalleled observability, and testability, all while fostering collaboration across the enterprise. With enterprise-grade hosting on Dagster Cloud, you gain even more capabilities, adding cost management, security, and CI support to further boost your teams' productivity. Go to [dagster.io](https://dagster.io/lp/dagster-cloud-trial?source=data-eng-podcast) today to get your first 30 days free! Support Data Engineering Podcast…
D
Data Engineering Podcast

Summary Working with data is a complicated process, with numerous chances for something to go wrong. Identifying and accounting for those errors is a critical piece of building trust in the organization that your data is accurate and up to date. While there are numerous products available to provide that visibility, they all have different technologies and workflows that they focus on. To bring observability to dbt projects the team at Elementary embedded themselves into the workflow. In this episode Maayan Salom explores the approach that she has taken to bring observability, enhanced testing capabilities, and anomaly detection into every step of the dbt developer experience. Announcements Hello and welcome to the Data Engineering Podcast, the show about modern data management Data lakes are notoriously complex. For data engineers who battle to build and scale high quality data workflows on the data lake, Starburst powers petabyte-scale SQL analytics fast, at a fraction of the cost of traditional methods, so that you can meet all your data needs ranging from AI to data applications to complete analytics. Trusted by teams of all sizes, including Comcast and Doordash, Starburst is a data lake analytics platform that delivers the adaptability and flexibility a lakehouse ecosystem promises. And Starburst does all of this on an open architecture with first-class support for Apache Iceberg, Delta Lake and Hudi, so you always maintain ownership of your data. Want to see Starburst in action? Go to dataengineeringpodcast.com/starburst and get $500 in credits to try Starburst Galaxy today, the easiest and fastest way to get started using Trino. Dagster offers a new approach to building and running data platforms and data pipelines. It is an open-source, cloud-native orchestrator for the whole development lifecycle, with integrated lineage and observability, a declarative programming model, and best-in-class testability. Your team can get up and running in minutes thanks to Dagster Cloud, an enterprise-class hosted solution that offers serverless and hybrid deployments, enhanced security, and on-demand ephemeral test deployments. Go to dataengineeringpodcast.com/dagster today to get started. Your first 30 days are free! This episode is brought to you by Datafold – a testing automation platform for data engineers that prevents data quality issues from entering every part of your data workflow, from migration to dbt deployment. Datafold has recently launched data replication testing, providing ongoing validation for source-to-target replication. Leverage Datafold's fast cross-database data diffing and Monitoring to test your replication pipelines automatically and continuously. Validate consistency between source and target at any scale, and receive alerts about any discrepancies. Learn more about Datafold by visiting dataengineeringpodcast.com/datafold . Your host is Tobias Macey and today I'm interviewing Maayan Salom about how to incorporate observability into a dbt-oriented workflow and how Elementary can help Interview Introduction How did you get involved in the area of data management? Can you start by outlining what elements of observability are most relevant for dbt projects? What are some of the common ad-hoc/DIY methods that teams develop to acquire those insights? What are the challenges/shortcomings associated with those approaches? Over the past ~3 years there were numerous data observability systems/products created. What are some of the ways that the specifics of dbt workflows are not covered by those generalized tools? What are the insights that can be more easily generated by embedding into the dbt toolchain and development cycle? Can you describe what Elementary is and how it is designed to enhance the development and maintenance work in dbt projects? How is Elementary designed/implemented? How have the scope and goals of the project changed since you started working on it? What are the engineering challenges/frustrations that you have dealt with in the creation and evolution of Elementary? Can you talk us through the setup and workflow for teams adopting Elementary in their dbt projects? How does the incorporation of Elementary change the development habits of the teams who are using it? What are the most interesting, innovative, or unexpected ways that you have seen Elementary used? What are the most interesting, unexpected, or challenging lessons that you have learned while working on Elementary? When is Elementary the wrong choice? What do you have planned for the future of Elementary? Contact Info LinkedIn Parting Question From your perspective, what is the biggest gap in the tooling or technology for data management today? Closing Announcements Thank you for listening! Don't forget to check out our other shows. Podcast.__init__ covers the Python language, its community, and the innovative ways it is being used. The Machine Learning Podcast helps you go from idea to production with machine learning. Visit the site to subscribe to the show, sign up for the mailing list, and read the show notes. If you've learned something or tried out a project from the show then tell us about it! Email hosts@dataengineeringpodcast.com ) with your story. Links Elementary Data Observability dbt Datadog pre-commit dbt packages SQLMesh Malloy SDF The intro and outro music is from The Hug by The Freak Fandango Orchestra / CC BY-SA Sponsored By: Starburst : 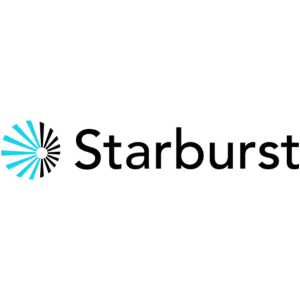 This episode is brought to you by Starburst - a data lake analytics platform for data engineers who are battling to build and scale high quality data pipelines on the data lake. Powered by Trino, Starburst runs petabyte-scale SQL analytics fast at a fraction of the cost of traditional methods, helping you meet all your data needs ranging from AI/ML workloads to data applications to complete analytics. Trusted by the teams at Comcast and Doordash, Starburst delivers the adaptability and flexibility a lakehouse ecosystem promises, while providing a single point of access for your data and all your data governance allowing you to discover, transform, govern, and secure all in one place. Starburst does all of this on an open architecture with first-class support for Apache Iceberg, Delta Lake and Hudi, so you always maintain ownership of your data. Want to see Starburst in action? Try Starburst Galaxy today, the easiest and fastest way to get started using Trino, and get $500 of credits free. [dataengineeringpodcast.com/starburst](https://www.dataengineeringpodcast.com/starburst) Datafold : 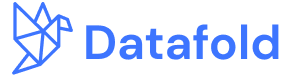 This episode is brought to you by Datafold – a testing automation platform for data engineers that prevents data quality issues from entering every part of your data workflow, from migration to dbt deployment. Datafold has recently launched data replication testing, providing ongoing validation for source-to-target replication. Leverage Datafold's fast cross-database data diffing and Monitoring to test your replication pipelines automatically and continuously. Validate consistency between source and target at any scale, and receive alerts about any discrepancies. Learn more about Datafold by visiting https://get.datafold.com/replication-de-podcast. Dagster : 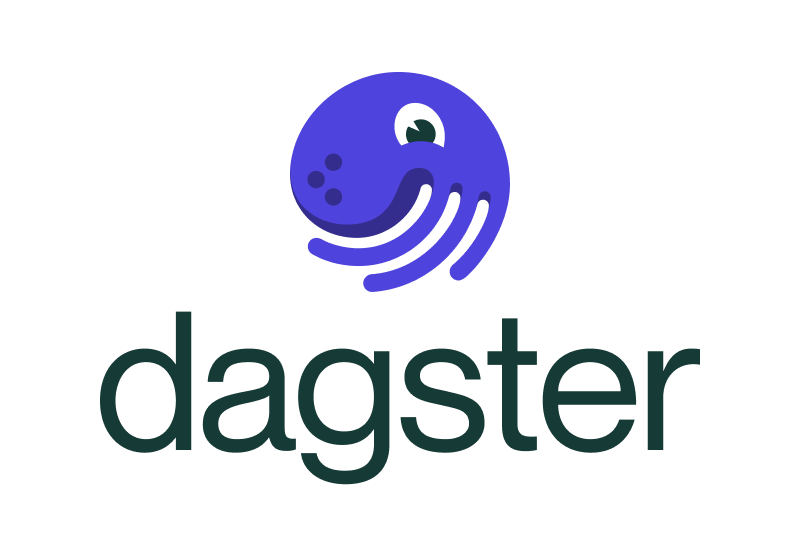 Data teams are tasked with helping organizations deliver on the premise of data, and with ML and AI maturing rapidly, expectations have never been this high. However data engineers are challenged by both technical complexity and organizational complexity, with heterogeneous technologies to adopt, multiple data disciplines converging, legacy systems to support, and costs to manage. Dagster is an open-source orchestration solution that helps data teams reign in this complexity and build data platforms that provide unparalleled observability, and testability, all while fostering collaboration across the enterprise. With enterprise-grade hosting on Dagster Cloud, you gain even more capabilities, adding cost management, security, and CI support to further boost your teams' productivity. Go to [dagster.io](https://dagster.io/lp/dagster-cloud-trial?source=data-eng-podcast) today to get your first 30 days free! Support Data Engineering Podcast…
D
Data Engineering Podcast

Summary A core differentiator of Dagster in the ecosystem of data orchestration is their focus on software defined assets as a means of building declarative workflows. With their launch of Dagster+ as the redesigned commercial companion to the open source project they are investing in that capability with a suite of new features. In this episode Pete Hunt, CEO of Dagster labs, outlines these new capabilities, how they reduce the burden on data teams, and the increased collaboration that they enable across teams and business units. Announcements Hello and welcome to the Data Engineering Podcast, the show about modern data management Dagster offers a new approach to building and running data platforms and data pipelines. It is an open-source, cloud-native orchestrator for the whole development lifecycle, with integrated lineage and observability, a declarative programming model, and best-in-class testability. Your team can get up and running in minutes thanks to Dagster Cloud, an enterprise-class hosted solution that offers serverless and hybrid deployments, enhanced security, and on-demand ephemeral test deployments. Go to dataengineeringpodcast.com/dagster today to get started. Your first 30 days are free! Data lakes are notoriously complex. For data engineers who battle to build and scale high quality data workflows on the data lake, Starburst powers petabyte-scale SQL analytics fast, at a fraction of the cost of traditional methods, so that you can meet all your data needs ranging from AI to data applications to complete analytics. Trusted by teams of all sizes, including Comcast and Doordash, Starburst is a data lake analytics platform that delivers the adaptability and flexibility a lakehouse ecosystem promises. And Starburst does all of this on an open architecture with first-class support for Apache Iceberg, Delta Lake and Hudi, so you always maintain ownership of your data. Want to see Starburst in action? Go to dataengineeringpodcast.com/starburst and get $500 in credits to try Starburst Galaxy today, the easiest and fastest way to get started using Trino. Your host is Tobias Macey and today I'm interviewing Pete Hunt about how the launch of Dagster+ will level up your data platform and orchestrate across language platforms Interview Introduction How did you get involved in the area of data management? Can you describe what the focus of Dagster+ is and the story behind it? What problems are you trying to solve with Dagster+? What are the notable enhancements beyond the Dagster Core project that this updated platform provides? How is it different from the current Dagster Cloud product? In the launch announcement you tease new capabilities that would be great to explore in turns: Make data a team sport, enabling data teams across the organization Deliver reliable, high quality data the organization can trust Observe and manage data platform costs Master the heterogeneous collection of technologies—both traditional and Modern Data Stack What are the business/product goals that you are focused on improving with the launch of Dagster+ What are the most interesting, innovative, or unexpected ways that you have seen Dagster used? What are the most interesting, unexpected, or challenging lessons that you have learned while working on the design and launch of Dagster+? When is Dagster+ the wrong choice? What do you have planned for the future of Dagster/Dagster Cloud/Dagster+? Contact Info Twitter LinkedIn Parting Question From your perspective, what is the biggest gap in the tooling or technology for data management today? Closing Announcements Thank you for listening! Don't forget to check out our other shows. Podcast.__init__ covers the Python language, its community, and the innovative ways it is being used. The Machine Learning Podcast helps you go from idea to production with machine learning. Visit the site to subscribe to the show, sign up for the mailing list, and read the show notes. If you've learned something or tried out a project from the show then tell us about it! Email hosts@dataengineeringpodcast.com ) with your story. Links Dagster Podcast Episode Dagster+ Launch Event Hadoop MapReduce Pydantic Software Defined Assets Dagster Insights Dagster Pipes Conway's Law Data Mesh Dagster Code Locations Dagster Asset Checks Dave & Buster's SQLMesh Podcast Episode SDF Malloy The intro and outro music is from The Hug by The Freak Fandango Orchestra / CC BY-SA Sponsored By: Starburst : 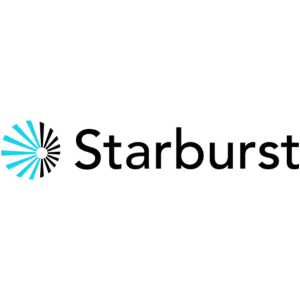 This episode is brought to you by Starburst - a data lake analytics platform for data engineers who are battling to build and scale high quality data pipelines on the data lake. Powered by Trino, Starburst runs petabyte-scale SQL analytics fast at a fraction of the cost of traditional methods, helping you meet all your data needs ranging from AI/ML workloads to data applications to complete analytics. Trusted by the teams at Comcast and Doordash, Starburst delivers the adaptability and flexibility a lakehouse ecosystem promises, while providing a single point of access for your data and all your data governance allowing you to discover, transform, govern, and secure all in one place. Starburst does all of this on an open architecture with first-class support for Apache Iceberg, Delta Lake and Hudi, so you always maintain ownership of your data. Want to see Starburst in action? Try Starburst Galaxy today, the easiest and fastest way to get started using Trino, and get $500 of credits free. [dataengineeringpodcast.com/starburst](https://www.dataengineeringpodcast.com/starburst) Dagster : 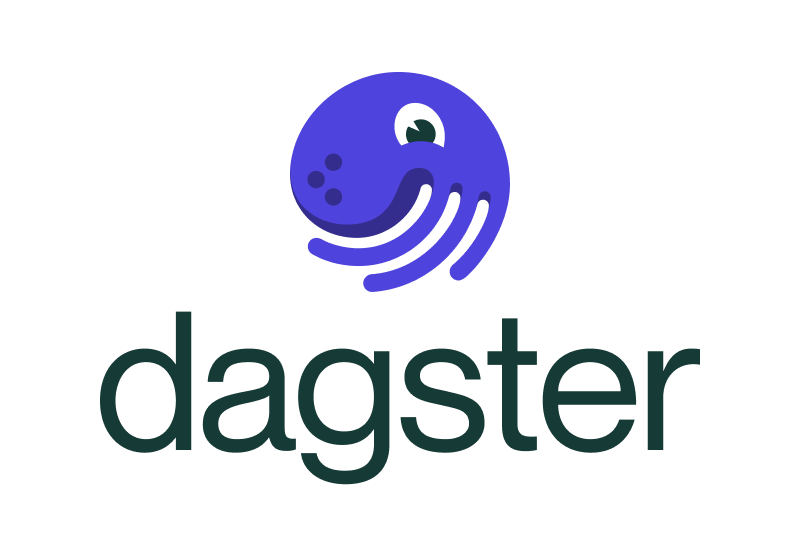 Data teams are tasked with helping organizations deliver on the premise of data, and with ML and AI maturing rapidly, expectations have never been this high. However data engineers are challenged by both technical complexity and organizational complexity, with heterogeneous technologies to adopt, multiple data disciplines converging, legacy systems to support, and costs to manage. Dagster is an open-source orchestration solution that helps data teams reign in this complexity and build data platforms that provide unparalleled observability, and testability, all while fostering collaboration across the enterprise. With enterprise-grade hosting on Dagster Cloud, you gain even more capabilities, adding cost management, security, and CI support to further boost your teams' productivity. Go to [dagster.io](https://dagster.io/lp/dagster-cloud-trial?source=data-eng-podcast) today to get your first 30 days free! Support Data Engineering Podcast…
欢迎使用Player FM
Player FM正在网上搜索高质量的播客,以便您现在享受。它是最好的播客应用程序,适用于安卓、iPhone和网络。注册以跨设备同步订阅。