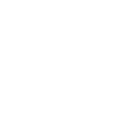
使用Player FM应用程序离线!
S2E29 - "Synthetic Data in AI: Challenges, Techniques & Use Cases" with Andrew Clark and Sid Mangalik (Monitaur)
Manage episode 377936032 series 3407760
This week I welcome Dr. Andrew Clark, Co-founder & CTO of Monitaur, a trusted domain expert on the topic of machine learning, auditing and assurance; and Sid Mangalik, Research Scientist at Monitaur and PhD student at Stony Brook University. I discovered Andrew and Sid's new podcast show, The AI Fundamentalists Podcast. I very much enjoyed their lively episode on Synthetic Data & AI, and am delighted to introduce them to my audience of privacy engineers.
In our conversation, we explore why data scientists must stress test their model validations, especially for consequential systems that affect human safety and reliability. In fact, we have much to learn from the aerospace engineering field who has been using ML/AI since the 1960s. We discuss the best and worst use cases for using synthetic data'; problems with LLM-generated synthetic data; what can go wrong when your AI models lack diversity; how to build fair, performant systems; & synthetic data techniques for use with AI.
Topics Covered:
- What inspired Andrew to found Monitaur and focus on AI governance
- Sid’s career path and his current PhD focus on NLP
- What motivated Andrew & Sid to launch their podcast, The AI Fundamentalists
- Defining 'synthetic data' & why academia takes a more rigorous approach to synthetic data than industry
- Whether the output of LLMs are synthetic data & the problem with training LLM base models with this data
- The best and worst 'synthetic data' use cases for ML/AI
- Why the 'quality' of input data is so important when training AI models
- Thoughts on OpenAI's announcement that it will use LLM-generated synthetic data; and critique of OpenAI's approach, the AI hype machine, and the problems with 'growth hacking' corner-cutting
- The importance of diversity when training AI models; using 'multi-objective modeling' for building fair & performant systems
- Andrew unpacks the "fairness through unawareness fallacy"
- How 'randomized data' differs from 'synthetic data'
- 4 techniques for using synthetic data with ML/AI: 1) the Monte Carlo method; 2) Latin hypercube sampling; 3) gaussian copulas; & 4) random walking
- What excites Andrew & Sid about synthetic data and how it will be used with AI in the future
Resources Mentioned:
- Check out Podchaser
- Listen to The AI Fundamentalists Podcast
- Check out Monitaur
Guest Info:
- Follow Andrew on LinkedIn
- Follow Sid on LinkedIn
Privado.ai
Privacy assurance at the speed of product development. Get instant visibility w/ privacy code scans.
Shifting Privacy Left Media
Where privacy engineers gather, share, & learn
Disclaimer: This post contains affiliate links. If you make a purchase, I may receive a commission at no extra cost to you.
Copyright © 2022 - 2024 Principled LLC. All rights reserved.
章节
1. S2E29 - "Synthetic Data in AI: Challenges, Techniques & Use Cases" with Andrew Clark and Sid Mangalik (Monitaur) (00:00:00)
2. Introducing Andrew Clark & Sid Mangalik (00:01:47)
3. What motivated Andrew to found Monitaur and focus on AI governance (00:04:06)
4. Sid shares his career path, why he chose to focus on AI governance, and how he ended up at Monitaur (00:09:09)
5. What motivated Andrew & Sid to launch their own podcast show, The AI Fundamentalists, & their intended audience (00:11:45)
6. The definition of 'synthetic data' and why academia takes a more rigorous approach to deploying and testing synthetic data than industry does (00:14:34)
7. Whether the output of LLMs are synthetic data and the problem with continuing to train LLM base models with this data (00:16:47)
8. What 'synthetic data' use cases are most helpful when it comes to AI, and which ones are the most unhelpful? (00:22:25)
9. Andrew & Sid discuss why the 'quality' of input data is so important for training AI models; and discussion of OpenAI's announcement that it plans to use LLM-generated synthetic data (00:26:50)
10. Andrew & Sid critique OpenAI's approach, the AI hype machine, and the problems with cutting corners via 'growth hacking' (00:29:39)
11. Andrew emphasizes the importance of diversity when training AI models and using 'multi-objective modeling' (00:33:34)
12. Andrew unpacks the "fairness through unawareness fallacy" for us (00:41:44)
13. Sid explains the difference between using 'randomized data' and 'synthetic data' with a fun example (00:44:18)
14. Andrew & Sid describe 4 techniques for using synthetic data with ML/AI: 1) the Monte Carlo method; 2) Latin hypercube sampling; 3) gaussian copulas; & 4) random walking (00:45:02)
15. Andrew & Sid describe what they are each most excited about when it comes to synthetic data and how it will be used in the future (00:50:37)
63集单集
Manage episode 377936032 series 3407760
This week I welcome Dr. Andrew Clark, Co-founder & CTO of Monitaur, a trusted domain expert on the topic of machine learning, auditing and assurance; and Sid Mangalik, Research Scientist at Monitaur and PhD student at Stony Brook University. I discovered Andrew and Sid's new podcast show, The AI Fundamentalists Podcast. I very much enjoyed their lively episode on Synthetic Data & AI, and am delighted to introduce them to my audience of privacy engineers.
In our conversation, we explore why data scientists must stress test their model validations, especially for consequential systems that affect human safety and reliability. In fact, we have much to learn from the aerospace engineering field who has been using ML/AI since the 1960s. We discuss the best and worst use cases for using synthetic data'; problems with LLM-generated synthetic data; what can go wrong when your AI models lack diversity; how to build fair, performant systems; & synthetic data techniques for use with AI.
Topics Covered:
- What inspired Andrew to found Monitaur and focus on AI governance
- Sid’s career path and his current PhD focus on NLP
- What motivated Andrew & Sid to launch their podcast, The AI Fundamentalists
- Defining 'synthetic data' & why academia takes a more rigorous approach to synthetic data than industry
- Whether the output of LLMs are synthetic data & the problem with training LLM base models with this data
- The best and worst 'synthetic data' use cases for ML/AI
- Why the 'quality' of input data is so important when training AI models
- Thoughts on OpenAI's announcement that it will use LLM-generated synthetic data; and critique of OpenAI's approach, the AI hype machine, and the problems with 'growth hacking' corner-cutting
- The importance of diversity when training AI models; using 'multi-objective modeling' for building fair & performant systems
- Andrew unpacks the "fairness through unawareness fallacy"
- How 'randomized data' differs from 'synthetic data'
- 4 techniques for using synthetic data with ML/AI: 1) the Monte Carlo method; 2) Latin hypercube sampling; 3) gaussian copulas; & 4) random walking
- What excites Andrew & Sid about synthetic data and how it will be used with AI in the future
Resources Mentioned:
- Check out Podchaser
- Listen to The AI Fundamentalists Podcast
- Check out Monitaur
Guest Info:
- Follow Andrew on LinkedIn
- Follow Sid on LinkedIn
Privado.ai
Privacy assurance at the speed of product development. Get instant visibility w/ privacy code scans.
Shifting Privacy Left Media
Where privacy engineers gather, share, & learn
Disclaimer: This post contains affiliate links. If you make a purchase, I may receive a commission at no extra cost to you.
Copyright © 2022 - 2024 Principled LLC. All rights reserved.
章节
1. S2E29 - "Synthetic Data in AI: Challenges, Techniques & Use Cases" with Andrew Clark and Sid Mangalik (Monitaur) (00:00:00)
2. Introducing Andrew Clark & Sid Mangalik (00:01:47)
3. What motivated Andrew to found Monitaur and focus on AI governance (00:04:06)
4. Sid shares his career path, why he chose to focus on AI governance, and how he ended up at Monitaur (00:09:09)
5. What motivated Andrew & Sid to launch their own podcast show, The AI Fundamentalists, & their intended audience (00:11:45)
6. The definition of 'synthetic data' and why academia takes a more rigorous approach to deploying and testing synthetic data than industry does (00:14:34)
7. Whether the output of LLMs are synthetic data and the problem with continuing to train LLM base models with this data (00:16:47)
8. What 'synthetic data' use cases are most helpful when it comes to AI, and which ones are the most unhelpful? (00:22:25)
9. Andrew & Sid discuss why the 'quality' of input data is so important for training AI models; and discussion of OpenAI's announcement that it plans to use LLM-generated synthetic data (00:26:50)
10. Andrew & Sid critique OpenAI's approach, the AI hype machine, and the problems with cutting corners via 'growth hacking' (00:29:39)
11. Andrew emphasizes the importance of diversity when training AI models and using 'multi-objective modeling' (00:33:34)
12. Andrew unpacks the "fairness through unawareness fallacy" for us (00:41:44)
13. Sid explains the difference between using 'randomized data' and 'synthetic data' with a fun example (00:44:18)
14. Andrew & Sid describe 4 techniques for using synthetic data with ML/AI: 1) the Monte Carlo method; 2) Latin hypercube sampling; 3) gaussian copulas; & 4) random walking (00:45:02)
15. Andrew & Sid describe what they are each most excited about when it comes to synthetic data and how it will be used in the future (00:50:37)
63集单集
所有剧集
×欢迎使用Player FM
Player FM正在网上搜索高质量的播客,以便您现在享受。它是最好的播客应用程序,适用于安卓、iPhone和网络。注册以跨设备同步订阅。