使用Player FM应用程序离线!
Labeling, transforming, and structuring training data sets for machine learning
Manage episode 248276630 series 61203
In this episode of the Data Show, I speak with Alex Ratner, project lead for Stanford’s Snorkel open source project; Ratner also recently garnered a faculty position at the University of Washington and is currently working on a company supporting and extending the Snorkel project. Snorkel is a framework for building and managing training data. Based on our survey from earlier this year, labeled data remains a key bottleneck for organizations building machine learning applications and services.
Ratner was a guest on the podcast a little over two years ago when Snorkel was a relatively new project. Since then, Snorkel has added more features, expanded into computer vision use cases, and now boasts many users, including Google, Intel, IBM, and other organizations. Along with his thesis advisor professor Chris Ré of Stanford, Ratner and his collaborators have long championed the importance of building tools aimed squarely at helping teams build and manage training data. With today’s release of Snorkel version 0.9, we are a step closer to having a framework that enables the programmatic creation of training data sets.
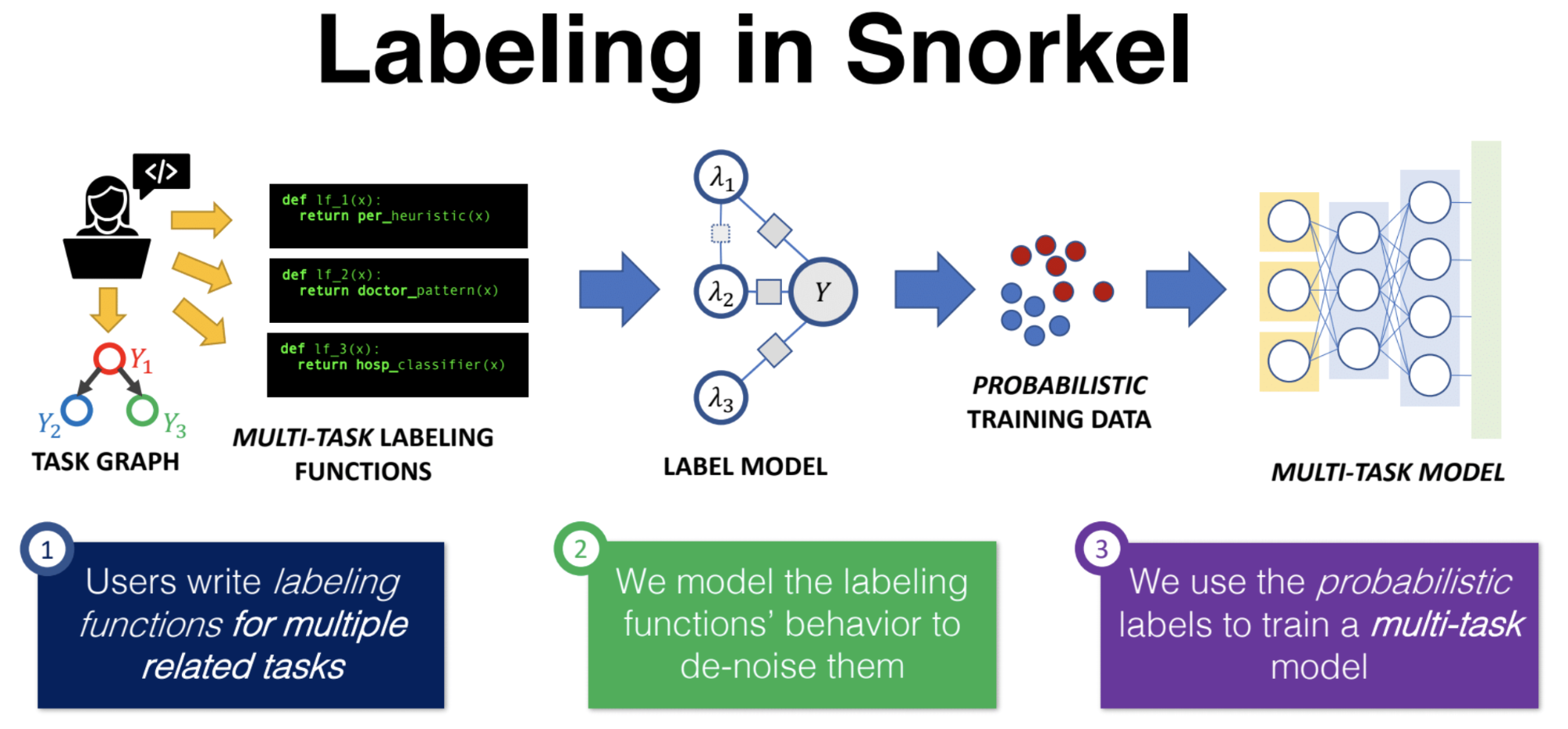
We had a great conversation spanning many topics, including:
- Why he and his collaborators decided to focus on “data programming” and tools for building and managing training data.
- A tour through Snorkel, including its target users and key components.
- What’s in the newly released version (v 0.9) of Snorkel.
- The number of Snorkel’s users has grown quite a bit since we last spoke, so we went through some of the common use cases for the project.
- Data lineage, AutoML, and end-to-end automation of machine learning pipelines.
- Holoclean and other projects focused on data quality and data programming.
- The need for tools that can ease the transition from raw data to derived data (e.g., entities), insights, and even knowledge.
Related resources:
- “Product management in the machine learning era”: A tutorial at the Artificial Intelligence Conference in San Jose, September 9-12, 2019.
- Chris Ré: “Software 2.0 and Snorkel”
- Alex Ratner: “Creating large training data sets quickly”
- Ihab Ilyas and Ben Lorica on “The quest for high-quality data”
- Roger Chen: “Acquiring and sharing high-quality data”
- Jeff Jonas on “Real-time entity resolution made accessible”
- “Data collection and data markets in the age of privacy and machine learning”
168集单集
Manage episode 248276630 series 61203
In this episode of the Data Show, I speak with Alex Ratner, project lead for Stanford’s Snorkel open source project; Ratner also recently garnered a faculty position at the University of Washington and is currently working on a company supporting and extending the Snorkel project. Snorkel is a framework for building and managing training data. Based on our survey from earlier this year, labeled data remains a key bottleneck for organizations building machine learning applications and services.
Ratner was a guest on the podcast a little over two years ago when Snorkel was a relatively new project. Since then, Snorkel has added more features, expanded into computer vision use cases, and now boasts many users, including Google, Intel, IBM, and other organizations. Along with his thesis advisor professor Chris Ré of Stanford, Ratner and his collaborators have long championed the importance of building tools aimed squarely at helping teams build and manage training data. With today’s release of Snorkel version 0.9, we are a step closer to having a framework that enables the programmatic creation of training data sets.
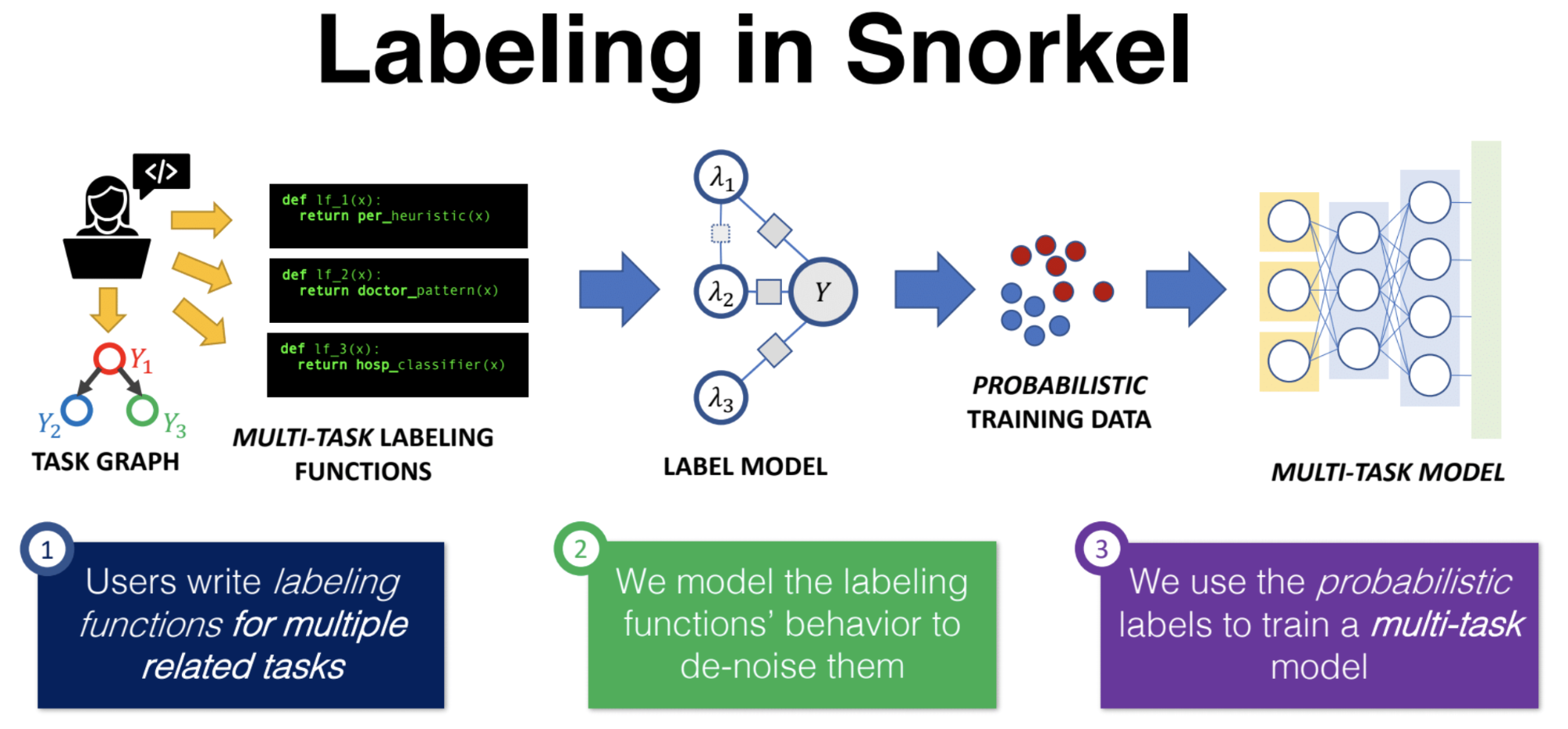
We had a great conversation spanning many topics, including:
- Why he and his collaborators decided to focus on “data programming” and tools for building and managing training data.
- A tour through Snorkel, including its target users and key components.
- What’s in the newly released version (v 0.9) of Snorkel.
- The number of Snorkel’s users has grown quite a bit since we last spoke, so we went through some of the common use cases for the project.
- Data lineage, AutoML, and end-to-end automation of machine learning pipelines.
- Holoclean and other projects focused on data quality and data programming.
- The need for tools that can ease the transition from raw data to derived data (e.g., entities), insights, and even knowledge.
Related resources:
- “Product management in the machine learning era”: A tutorial at the Artificial Intelligence Conference in San Jose, September 9-12, 2019.
- Chris Ré: “Software 2.0 and Snorkel”
- Alex Ratner: “Creating large training data sets quickly”
- Ihab Ilyas and Ben Lorica on “The quest for high-quality data”
- Roger Chen: “Acquiring and sharing high-quality data”
- Jeff Jonas on “Real-time entity resolution made accessible”
- “Data collection and data markets in the age of privacy and machine learning”
168集单集
所有剧集
×欢迎使用Player FM
Player FM正在网上搜索高质量的播客,以便您现在享受。它是最好的播客应用程序,适用于安卓、iPhone和网络。注册以跨设备同步订阅。