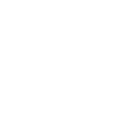
使用Player FM应用程序离线!
Boost Your Model: Wie funktioniert Data-Science im Maschinenraum?
Manage episode 452217722 series 3394916
Summary: In this conversation, Aleksander Fegel and Dr. David Geisel discuss the critical aspects of feature selection and engineering in data science. They explore the importance of understanding features, the role of data quality, and the necessity of engaging with domain experts. The discussion covers various methods of feature selection, including filter methods, wrapper methods, and embedded methods, emphasizing the need for simplicity and clarity in model building. The conversation concludes with insights on how to effectively interpret model predictions and the significance of collaboration in data science projects.
Takeaways: -Feature selection is essential for successful data modeling. -Data quality significantly impacts model performance. -Engaging with domain experts enhances feature selection. -Simplicity in models often leads to better results. -Feature importance helps in understanding model predictions. -Different methods exist for feature selection, each with pros and cons. -Collaboration between data scientists and domain experts is crucial. -Feature engineering is a continuous process in data science. -Understanding the context of data is vital for effective modeling. -Iterative testing and validation are key to successful feature selection. titles
20集单集
Manage episode 452217722 series 3394916
Summary: In this conversation, Aleksander Fegel and Dr. David Geisel discuss the critical aspects of feature selection and engineering in data science. They explore the importance of understanding features, the role of data quality, and the necessity of engaging with domain experts. The discussion covers various methods of feature selection, including filter methods, wrapper methods, and embedded methods, emphasizing the need for simplicity and clarity in model building. The conversation concludes with insights on how to effectively interpret model predictions and the significance of collaboration in data science projects.
Takeaways: -Feature selection is essential for successful data modeling. -Data quality significantly impacts model performance. -Engaging with domain experts enhances feature selection. -Simplicity in models often leads to better results. -Feature importance helps in understanding model predictions. -Different methods exist for feature selection, each with pros and cons. -Collaboration between data scientists and domain experts is crucial. -Feature engineering is a continuous process in data science. -Understanding the context of data is vital for effective modeling. -Iterative testing and validation are key to successful feature selection. titles
20集单集
所有剧集
×欢迎使用Player FM
Player FM正在网上搜索高质量的播客,以便您现在享受。它是最好的播客应用程序,适用于安卓、iPhone和网络。注册以跨设备同步订阅。